Dynamic service function chain arrangement method and system based on deep reinforcement learning
A service function chain and reinforcement learning technology, which is applied in the field of dynamic service function chain arrangement based on deep reinforcement learning, can solve the problem of limited edge cloud resources, local optimal solutions that are difficult to adapt to dynamic network changes, reasonable allocation and increased use of resources Difficulty and other issues, to achieve network load balance, improve the reception rate, avoid resource bottleneck nodes and links
- Summary
- Abstract
- Description
- Claims
- Application Information
AI Technical Summary
Problems solved by technology
Method used
Image
Examples
Embodiment 1
[0060] like figure 1 As shown, this embodiment discloses a method for orchestrating dynamic service function chains based on deep reinforcement learning, including the following steps:
[0061] S1. Obtain the historical network status according to the SDN controller; the network status includes the service function chain request flow information generated in the IoT network supporting mobile edge computing and the corresponding network resource status information;
[0062] Specifically, the service function chain request flow is the request flow sent by the IoT terminal in the IoT network that supports mobile edge computing, and the request flow needs to traverse different VNFs in a predefined order; the network resource status information includes The remaining rate of CPU computing resources, the remaining rate of bandwidth resources on the link, the processing delay of VNF instances on the micro-cloud, and the transmission delay of data traffic on the link.
[0063] S2. Se...
Embodiment 2
[0116] like image 3 As shown, this embodiment provides a dynamic service function chain orchestration system based on deep reinforcement learning, including:
[0117] The IoT terminal is used to generate service function chain request flow information supporting mobile edge computing in the IoT network;
[0118] SDN Controller (SDN Controller), used to obtain the service function chain request flow information and network resource status information; and be responsible for the dynamic deployment of VNF on the micro-cloud and the configuration of management service function chain (SFC) request flow routing path .
[0119] Agents include:
[0120] A predefined module for setting deep reinforcement learning parameters and initializing the weight of the neural network according to the obtained network state;
[0121] The network training module is used to train the neural network according to the experience samples generated by the interaction between the agent and the environ...
PUM
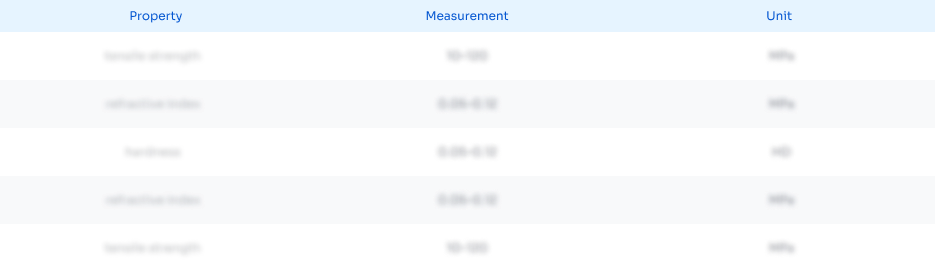
Abstract
Description
Claims
Application Information
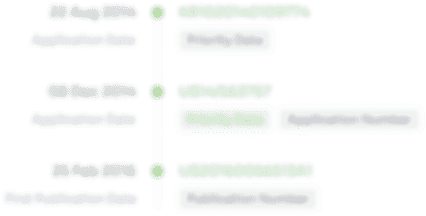
- R&D Engineer
- R&D Manager
- IP Professional
- Industry Leading Data Capabilities
- Powerful AI technology
- Patent DNA Extraction
Browse by: Latest US Patents, China's latest patents, Technical Efficacy Thesaurus, Application Domain, Technology Topic, Popular Technical Reports.
© 2024 PatSnap. All rights reserved.Legal|Privacy policy|Modern Slavery Act Transparency Statement|Sitemap|About US| Contact US: help@patsnap.com