Intermediate infrared spectrum rapid batch detection method for total solid content in buffalo milk and application of intermediate infrared spectrum rapid batch detection method
A technology of total solids and infrared spectroscopy, applied in measuring devices, material analysis through optical means, instruments, etc., can solve the problems of low accuracy and achieve high accuracy, rapid batch detection, and strong practicability
- Summary
- Abstract
- Description
- Claims
- Application Information
AI Technical Summary
Problems solved by technology
Method used
Image
Examples
Embodiment 1
[0051] Selection of predictive model algorithm for total solids content of buffalo milk:
[0052] The purpose of this application is to establish a quantitative prediction model for the total solid content of buffalo milk, so the modeling algorithm used is a regression algorithm. There are many kinds of regression algorithms. In this embodiment, Ridge regression (Ridge) and partial least squares regression (PLSR) algorithms are mainly used for model establishment and comparison. The reasons are as follows:
[0053] Ridge regression is a type of linear regression. Only when the algorithm establishes the regression equation, the ridge regression adds regularization restrictions, so as to achieve the effect of solving over-fitting. There are two types of regularization, namely l1 regularization and l2 regularization. Compared with l1 regularization, the advantages of l2 regularization are: (1) cross-validation can be performed (2) stochastic gradient descent is realized. Ridge ...
Embodiment 2
[0056] Choice of Quantitative Prediction Model Algorithm:
[0057] In this embodiment, each sample corresponds to one piece of MIR spectral data. Will be greater than 4000cm -1 Partially modeled and compared the accuracy of the analysis model without using any preprocessing method to determine the accuracy of the algorithm. The results are shown in the following table:
[0058] Algorithm comparison results:
[0059] algorithm R c
[0060] After comparing the results of the two algorithms, PLSR has a better effect on the test set and is close to the effect of the training set, so the PLSR algorithm is finally selected for modeling.
Embodiment 3
[0062] Establishment of a method for detecting total solids content in buffalo milk by mid-infrared spectroscopy:
[0063] 1. Division of modeling data sets
[0064]
[0065] In the division of the modeling data set in this embodiment, 80% is a training set and 20% is a test set. The ratio of the training set to the test set is 4:1. At the same time, the training set is also called the cross-validation set. In the process of training the model, 10-fold cross-validation is performed.
[0066] 2. Screening of modeling MIR data preprocessing methods
[0067] Effective feature screening is the basic operation of spectral data processing, the purpose is to eliminate noise and lay a good foundation for feature extraction. Effective feature screening mainly includes feature extraction, feature preprocessing, and feature dimensionality reduction. This embodiment mainly performs feature preprocessing on the spectral data. First, SG (convolution smoothing), MSC (multiple scatterin...
PUM
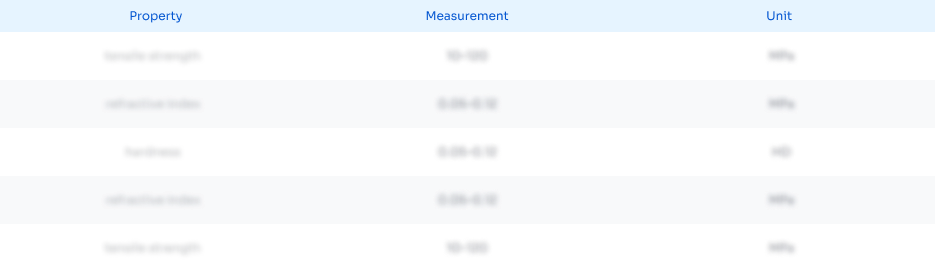
Abstract
Description
Claims
Application Information
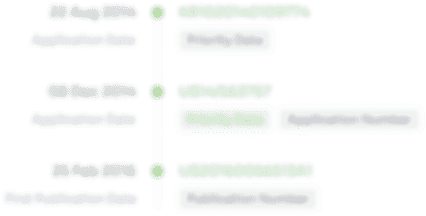
- R&D Engineer
- R&D Manager
- IP Professional
- Industry Leading Data Capabilities
- Powerful AI technology
- Patent DNA Extraction
Browse by: Latest US Patents, China's latest patents, Technical Efficacy Thesaurus, Application Domain, Technology Topic, Popular Technical Reports.
© 2024 PatSnap. All rights reserved.Legal|Privacy policy|Modern Slavery Act Transparency Statement|Sitemap|About US| Contact US: help@patsnap.com