Unsupervised decoupling image generation method based on invariant information distillation
An image generation, unsupervised technology, applied in the field of computer vision, can solve problems such as poor image effect and poor image generation quality, and achieve the effect of improving image decoupling generation effect, decoupling ability, and standardized mutual information
- Summary
- Abstract
- Description
- Claims
- Application Information
AI Technical Summary
Problems solved by technology
Method used
Image
Examples
Embodiment Construction
[0081] Step 1: Preprocessing the experimental data;
[0082] Get the CIFAR10 dataset from official sources. The CIFAR10 dataset is an image dataset composed of 60,000 color RGB images. The dataset contains 10 categories in total, and the number of images in each category is equal. In addition, the size of each image in the dataset is 32×32, and it is marked corresponding category information. In order to convert the image data into data that is easier for the deep learning model to learn, the image pixel values are normalized to the interval [-1,1] and converted into tensors for storage.
[0083] Step 2: Perform random data enhancement operations on experimental data;
[0084] To perform random data enhancement processing on the image data processed in step 1, a total of four operations including random cropping, random horizontal flip, random brightness change and random grayscale are used. The specific random data enhancement process for each image is as follows: the fi...
PUM
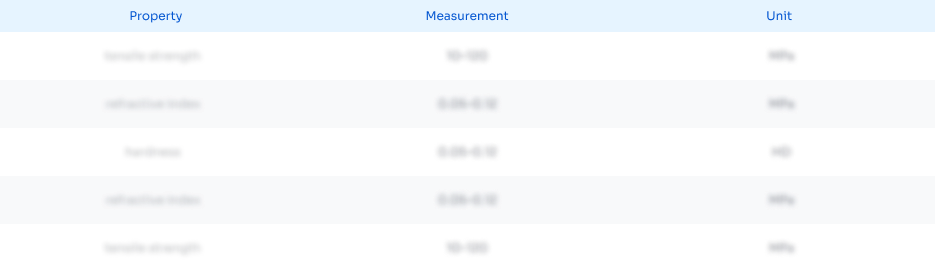
Abstract
Description
Claims
Application Information
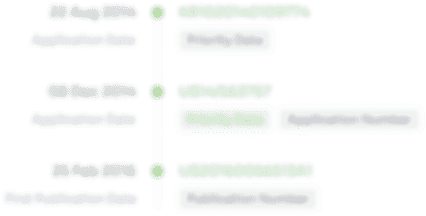
- R&D Engineer
- R&D Manager
- IP Professional
- Industry Leading Data Capabilities
- Powerful AI technology
- Patent DNA Extraction
Browse by: Latest US Patents, China's latest patents, Technical Efficacy Thesaurus, Application Domain, Technology Topic.
© 2024 PatSnap. All rights reserved.Legal|Privacy policy|Modern Slavery Act Transparency Statement|Sitemap