Comprehensive energy load prediction method based on multi-task learning strategy and deep learning
A multi-task learning and deep learning technology, applied in the field of comprehensive energy load forecasting based on multi-task learning strategies and deep learning, can solve undiscovered problems, achieve the effects of fewer models, improved load forecasting accuracy, and good forecasting results
- Summary
- Abstract
- Description
- Claims
- Application Information
AI Technical Summary
Problems solved by technology
Method used
Image
Examples
Embodiment Construction
[0029] Embodiments of the present invention are described in further detail below in conjunction with the accompanying drawings:
[0030] A comprehensive energy load forecasting method based on multi-task learning strategy and deep learning, comprising the following steps:
[0031] S1. Obtain the characteristics of the influencing factors considering the impact on the cooling, heating, and electric loads of the integrated energy system, and form a historical cooling, heating, and electrical load feature library and an influencing factor feature library;
[0032] The influencing factor characteristics in the step S1 include: meteorological factor characteristics and time factor characteristics closely related to cooling, heating and electrical loads.
[0033] The characteristics of the meteorological factors are analyzed through the Pearson correlation coefficient, that is, the Pearson correlation coefficients of the characteristics of the meteorological factors such as tempera...
PUM
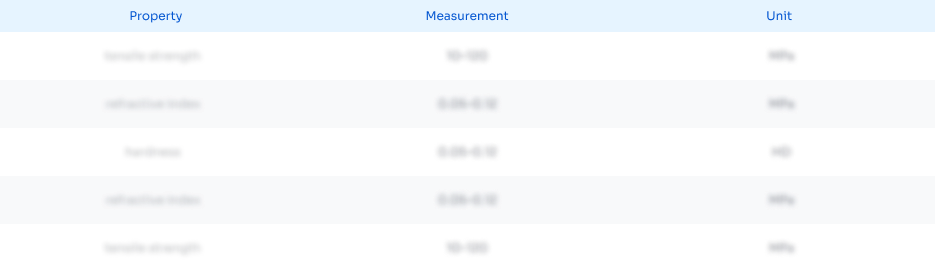
Abstract
Description
Claims
Application Information
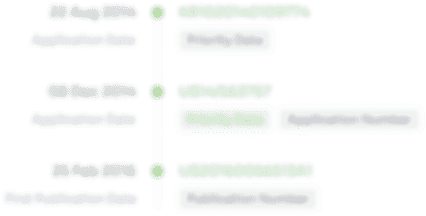
- R&D Engineer
- R&D Manager
- IP Professional
- Industry Leading Data Capabilities
- Powerful AI technology
- Patent DNA Extraction
Browse by: Latest US Patents, China's latest patents, Technical Efficacy Thesaurus, Application Domain, Technology Topic, Popular Technical Reports.
© 2024 PatSnap. All rights reserved.Legal|Privacy policy|Modern Slavery Act Transparency Statement|Sitemap|About US| Contact US: help@patsnap.com