Pre-training model-based domain map entity and relationship joint extraction method and system
A pre-training and model technology, applied in the field of big data, can solve problems such as the complicated process of map construction, ignoring the important characteristics of the joint, and the inability to achieve joint training, etc., to achieve the effect of reducing labor costs, reducing complexity, and being easy to understand
- Summary
- Abstract
- Description
- Claims
- Application Information
AI Technical Summary
Problems solved by technology
Method used
Image
Examples
Embodiment 1
[0066] refer to figure 1 , has illustrated the flow process of the method operation of the present invention.
[0067] The knowledge map entity and relation extraction method based on the pre-trained model described in this embodiment includes the following steps:
[0068] (1) Obtain the original data, divide the data into a training set and a test set after labeling, and establish a preliminary small-scale insurance labeling data set U and a candidate relationship set V, specifically including the following steps:
[0069] (1.1) Grab the text information in the insurance field on the relevant websites of the insurance company, use the crawler to grab the product introduction and comparative analysis of the specific insurance website, and finally save it in a unified text form;
[0070] (1.2) Data cleaning, to filter out key paragraphs from the acquired text, remove useless information such as head and tail, pictures, etc.; small-scale labeling, select some representative sen...
Embodiment 2
[0081] refer to figure 2 , is the model architecture used for map relationship and entity pair extraction, which can be divided into three specific modules:
[0082] (1) Pre-training model encoding module:
[0083] The pre-training model encoding module can effectively capture the contextual semantic information, and convert the sentence S=[w 1 ,...w n ], n represents the length of the sentence, as the input of the pre-training model to obtain the feature vector representation of the sentence sequence, in order to obtain the sentence w i The context of each token means x i , different Transformer-based networks can be used. In the present invention, the pre-training model BERT (not limited to BERT) is used as the basic encoder. The BERT output is as follows:
[0084] {x 1 ,...,x n} = BERTw 1 ,...,w n})
[0085] Here is consistent with the common one, the feature encoding x of each word in the sentence i The corresponding tag, segment and position information are sum...
Embodiment 3
[0100] refer to image 3 , is the proportion of each relationship in the finally extracted triplet data.
[0101] The original text is based on related products in the insurance field, and has strong pertinence. In the description of an insurance product, there are limited types of common relationships, and the model can achieve better results in actual extraction. finally showed image 3 proportion of the situation.
[0102] Among them, the most common relationship types are generally the first dozen or so, and the frequency of subsequent relationships is greatly reduced. All the remaining relationships with low frequency of occurrence are classified as "other" and the proportion is almost the same as that of the highest relationship. It can be seen that when constructing a map in a specific field, it is very likely that there will be a relatively concentrated relationship type, which will help researchers use the data for subsequent research and analysis.
PUM
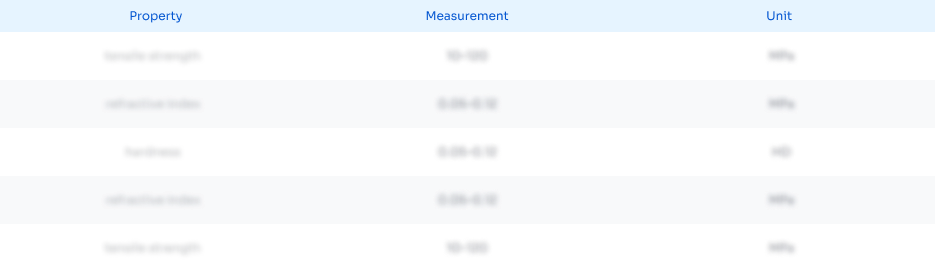
Abstract
Description
Claims
Application Information
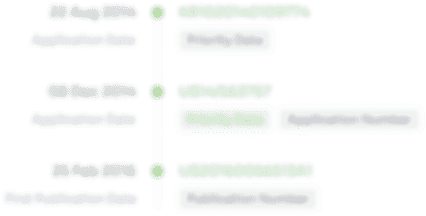
- R&D
- Intellectual Property
- Life Sciences
- Materials
- Tech Scout
- Unparalleled Data Quality
- Higher Quality Content
- 60% Fewer Hallucinations
Browse by: Latest US Patents, China's latest patents, Technical Efficacy Thesaurus, Application Domain, Technology Topic, Popular Technical Reports.
© 2025 PatSnap. All rights reserved.Legal|Privacy policy|Modern Slavery Act Transparency Statement|Sitemap|About US| Contact US: help@patsnap.com