Weighted bone age evaluation method and system based on deep learning
A technology of deep learning and bone age, applied in the weighted bone age assessment method and system based on deep learning, in the field of bone age assessment, can solve problems such as complexity, impact of assessment results, different assessment levels, etc., and achieve high accuracy
- Summary
- Abstract
- Description
- Claims
- Application Information
AI Technical Summary
Problems solved by technology
Method used
Image
Examples
Embodiment 1
[0058] As shown in the figure, Embodiment 1 of the present invention provides a weighted bone age assessment method based on deep learning.
[0059] The method includes:
[0060] Preprocessing the X-ray images of the tester's hand bones;
[0061] Roughly segment the preprocessed image to obtain the ROI sets corresponding to different metacarpal and phalanx bones and the ROI sets of the carpal and radius and ulnar bones;
[0062] Input multiple metacarpal and phalanx ROI sets and carpal, radius and ulna ROI sets into the pre-established hand bone fine segmentation model after rough segmentation, and obtain multiple hand bone ROIs after fine segmentation;
[0063] Input the regions of interest of multiple hand bones obtained after subdivision into the pre-established and trained hand bone classification and rating model to obtain the corresponding classification and development level of each hand bone;
[0064] According to the evaluation chart of the developmental maturity of...
Embodiment 2
[0121] Embodiment 2 of the present invention proposes a weighted bone age assessment system based on deep learning. The system includes: hand bone fine segmentation model, hand bone classification and rating model, preprocessing module, rough segmentation module, fine segmentation module, classification and rating Module, RUS-CHN evaluation module, TW3-C Carpal evaluation module and weighted output module; Concrete processing method is the same as embodiment 1, wherein,
[0122] The preprocessing module is used to preprocess the X-ray images of the tester's hand bones;
[0123] The rough segmentation module is used to roughly segment the preprocessed image, and respectively obtain the ROI sets corresponding to different metacarpal and phalanx and the carpal and radius and ulna ROI sets;
[0124] The fine-segmentation module is used to input a plurality of metacarpophalangeal ROI sets and carpal and radius-ulna ROI sets into the pre-established hand bone fine-segmentation model...
PUM
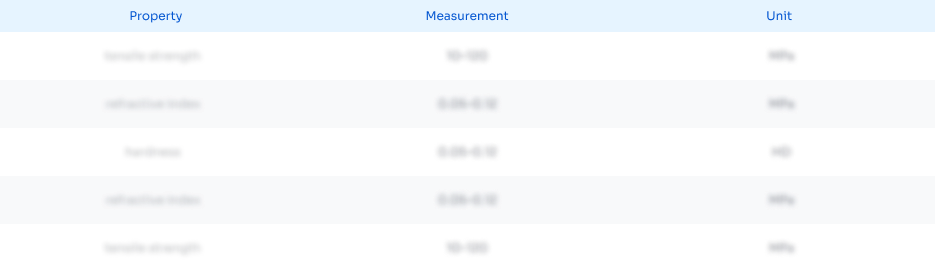
Abstract
Description
Claims
Application Information
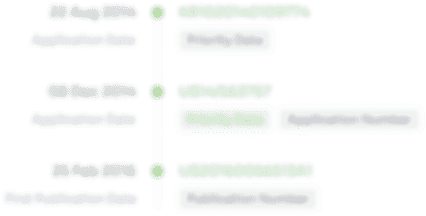
- Generate Ideas
- Intellectual Property
- Life Sciences
- Materials
- Tech Scout
- Unparalleled Data Quality
- Higher Quality Content
- 60% Fewer Hallucinations
Browse by: Latest US Patents, China's latest patents, Technical Efficacy Thesaurus, Application Domain, Technology Topic, Popular Technical Reports.
© 2025 PatSnap. All rights reserved.Legal|Privacy policy|Modern Slavery Act Transparency Statement|Sitemap|About US| Contact US: help@patsnap.com