Under-sampling lung gas MRI reconstruction method based on multi-task complex value deep learning
A deep learning and under-sampling technology, applied in the field of imaging, can solve the problems that affect the reconstruction results, the convolutional neural network is difficult to extract image features, and hyperpolarized gas MRI is easily affected by noise and artifacts, so as to improve the quality of reconstruction , good image details, and the effect of speeding up the imaging speed
- Summary
- Abstract
- Description
- Claims
- Application Information
AI Technical Summary
Problems solved by technology
Method used
Image
Examples
Embodiment Construction
[0044] In order to facilitate those of ordinary skill in the art to understand and implement the present invention, the present invention will be described in further detail below in conjunction with the examples. It should be understood that the implementation examples described here are only used to illustrate and explain the present invention, and are not intended to limit the present invention.
[0045] like figure 1 As shown, the flow chart of the undersampled lung gas MRI reconstruction method for multi-task complex-valued deep learning includes the following steps:
[0046] Step 1. Use 3D bSSFP sequence to scan 94 volunteers to obtain 3D full-sampled k-space data and corresponding under-sampled k-space data. The size of the 3D full-sampled k-space data matrix is 96×96, the number of layers is 24, and the acceleration factor is 4 Times, the sampling method is Cartesian sampling, the schematic diagram of the sampling matrix is as follows figure 2 shown. Extract 3D ...
PUM
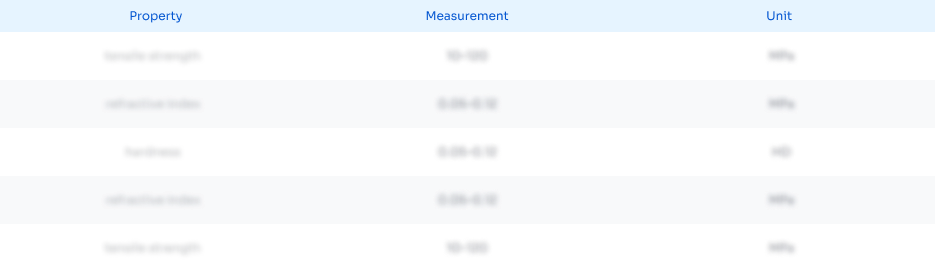
Abstract
Description
Claims
Application Information
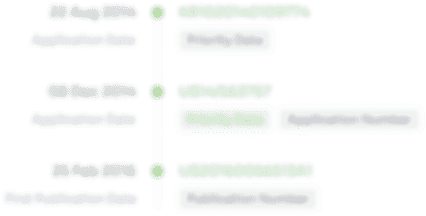
- R&D Engineer
- R&D Manager
- IP Professional
- Industry Leading Data Capabilities
- Powerful AI technology
- Patent DNA Extraction
Browse by: Latest US Patents, China's latest patents, Technical Efficacy Thesaurus, Application Domain, Technology Topic, Popular Technical Reports.
© 2024 PatSnap. All rights reserved.Legal|Privacy policy|Modern Slavery Act Transparency Statement|Sitemap|About US| Contact US: help@patsnap.com