Industrial part defect detection method based on deep learning
A technology of deep learning and defect detection, applied in neural learning methods, optical testing flaws/defects, computer components, etc., can solve problems such as large number of parameters, large data requirements, and inability to meet the real-time requirements of industrial assembly lines
- Summary
- Abstract
- Description
- Claims
- Application Information
AI Technical Summary
Problems solved by technology
Method used
Image
Examples
Embodiment 1
[0034] In order to solve the existing technical means, for the detection of industrial parts defects, the manual detection standards are not uniform, the continuity is low, the early learning time cost of modular intelligent detection is high, the parameter demand is large, and the problems cannot meet the new industrial speed requirements. The present invention proposes a new fusion neural network, so as to realize the high efficiency of the detection speed under the limited amount of parameters, and can ensure the resolution and clarity of the output defect position image. The details are as follows: figure 1 As shown, a method for detecting defects in industrial parts based on deep learning, including steps:
[0035] S1: Obtain a preset number of original images of industrial parts and defect-marked defect-marked images (images marked with defects in the original images of industrial parts);
[0036] S2: Obtain the feature map after convolution pooling processing according ...
PUM
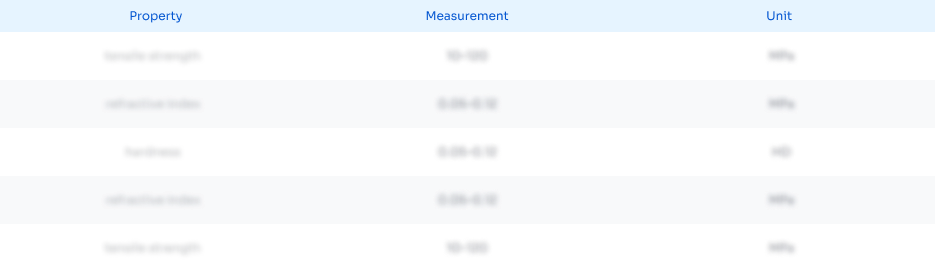
Abstract
Description
Claims
Application Information
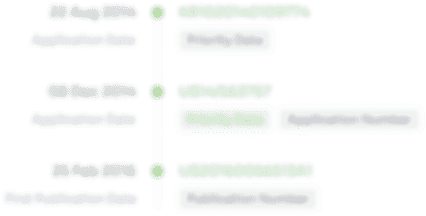
- R&D Engineer
- R&D Manager
- IP Professional
- Industry Leading Data Capabilities
- Powerful AI technology
- Patent DNA Extraction
Browse by: Latest US Patents, China's latest patents, Technical Efficacy Thesaurus, Application Domain, Technology Topic, Popular Technical Reports.
© 2024 PatSnap. All rights reserved.Legal|Privacy policy|Modern Slavery Act Transparency Statement|Sitemap|About US| Contact US: help@patsnap.com