Personalized federal learning method based on multi-head attention mechanism
A learning method and attention technology, applied in the fields of privacy protection and data security, can solve the problems of not taking into account the correlation of extracted features, not taking into account the differences of client data, etc.
- Summary
- Abstract
- Description
- Claims
- Application Information
AI Technical Summary
Problems solved by technology
Method used
Image
Examples
Embodiment Construction
[0037] The specific embodiment of the present invention is further described below in conjunction with accompanying drawing:
[0038] A personalized federated learning method based on multi-head attention mechanism, characterized in that: it is characterized in that it comprises the following steps:
[0039] Step 1: Build a local model of federated learning. Multi-head attention mechanism model: use the multi-head attention mechanism in the most classic convolutional neural network. Through the multi-head attention mechanism, key information is retained, feature extraction and selection are better, and the accuracy of identification;
[0040] Step 2: Build the multi-head attention mechanism model of the federated learning global model: Considering the impact of the personalization of each local model on the global model, when sending the global model parameters to the local model, make corresponding changes according to the personalized characteristics of the model .
[0041...
PUM
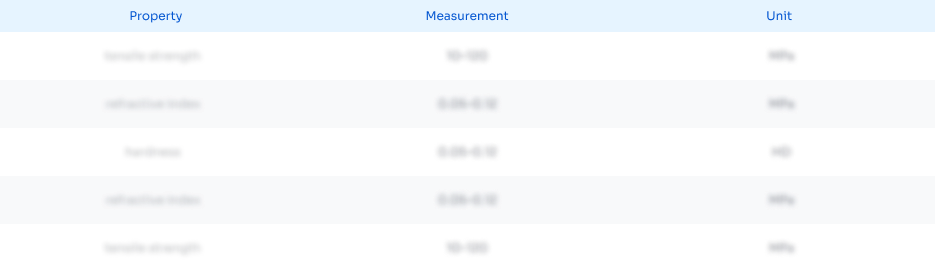
Abstract
Description
Claims
Application Information
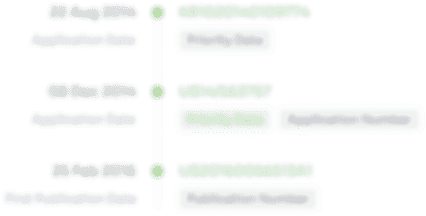
- R&D Engineer
- R&D Manager
- IP Professional
- Industry Leading Data Capabilities
- Powerful AI technology
- Patent DNA Extraction
Browse by: Latest US Patents, China's latest patents, Technical Efficacy Thesaurus, Application Domain, Technology Topic, Popular Technical Reports.
© 2024 PatSnap. All rights reserved.Legal|Privacy policy|Modern Slavery Act Transparency Statement|Sitemap|About US| Contact US: help@patsnap.com