A Monocular 3D Object Detection Method Based on Lightweight Feature Pyramid Structure
A feature pyramid and target detection technology, applied in neural learning methods, neural architecture, character and pattern recognition, etc., can solve problems that cannot fully meet perception needs, affect real-time deployment of algorithms, increase model reasoning delay, etc., and shorten the model Effects of reasoning delay, improving algorithm accuracy index, and overcoming algorithm efficiency
- Summary
- Abstract
- Description
- Claims
- Application Information
AI Technical Summary
Problems solved by technology
Method used
Image
Examples
Embodiment 1
[0102] The method in Embodiment 1 may be applied to or implemented by a processor. A processor may be an integrated circuit chip with signal processing capabilities. In the implementation process, each step of the above method can be completed by an integrated logic circuit of hardware in a processor or an instruction in the form of software. The above-mentioned processor may be a general-purpose processor, a digital signal processor (Digital Signal Processor, DSP), an application specific integrated circuit (Application Specific Integrated Circuit, ASIC), a field programmable gate array (Field Programmable Gate Array, FPGA) or other programmable Logic devices, discrete gate or transistor logic devices, discrete hardware components. The methods, steps and logic block diagrams disclosed in Embodiment 1 can be realized or executed. A general-purpose processor may be a microprocessor, or the processor may be any conventional processor, or the like. The steps of the method disc...
PUM
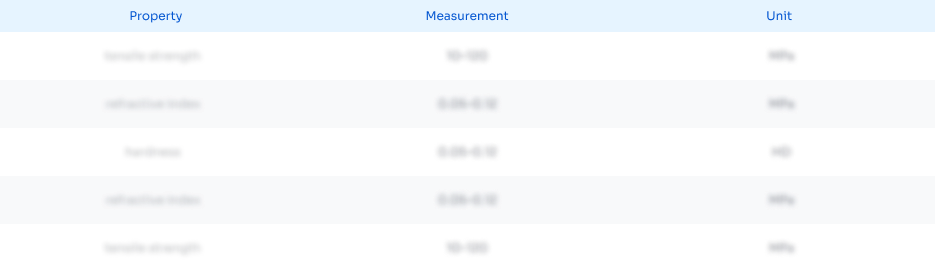
Abstract
Description
Claims
Application Information
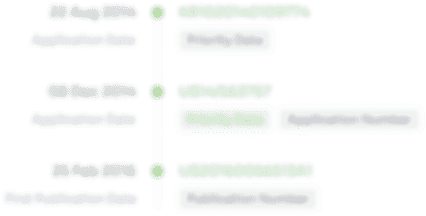
- Generate Ideas
- Intellectual Property
- Life Sciences
- Materials
- Tech Scout
- Unparalleled Data Quality
- Higher Quality Content
- 60% Fewer Hallucinations
Browse by: Latest US Patents, China's latest patents, Technical Efficacy Thesaurus, Application Domain, Technology Topic, Popular Technical Reports.
© 2025 PatSnap. All rights reserved.Legal|Privacy policy|Modern Slavery Act Transparency Statement|Sitemap|About US| Contact US: help@patsnap.com