Medical image classification method and device based on multi-view learning and depth supervision auto-encoder
A medical imaging and autoencoder technology, applied in image analysis, image enhancement, image data processing, etc., can solve problems such as parameter adjustment and function selection sensitivity
- Summary
- Abstract
- Description
- Claims
- Application Information
AI Technical Summary
Problems solved by technology
Method used
Image
Examples
Embodiment 1
[0075] see figure 1 The image classification method based on multi-morphological multi-task feature selection provided by this embodiment contains the following steps:
[0076] Step 1: Obtain the CT image of the subject (in this embodiment, CT is the image), and first perform preprocessing on the image of each subject, specifically: use the dcm2nii software package to convert the medical image of each case Reconstructed into a 3D image; then the 3D image was preprocessed using the 3D U-Net model to extract the lung parenchyma of the 3D image; in order to overcome the difference between sample thickness variations, the volumetric data of the lung parenchyma were resampled by B-spline interpolation Voxel resolution of 1mm x 1mm x 1mm.
[0077] Step 2: Perform wavelet decomposition on the region of interest of the medical image preprocessed in step 1 to obtain multi-frequency subbands;
[0078] Each segmented volume is textured using 3D-WT to capture eight distinct frequency su...
Embodiment 2
[0126] This embodiment discloses a medical image classification device based on multi-view learning and deep supervision autoencoder, including the following modules:
[0127] The image wavelet transform module is used to perform step 1: use wavelet transform to perform wavelet decomposition on the region of interest of each image, and then obtain multiple subbands in different frequency domains, and each subband is defined as a view.
[0128] The multi-view feature extraction module is used to perform step 2: quantitatively extract 93 morphological features for each view, and then obtain multi-view features;
[0129] The classifier construction and training module is used to perform step 3: construct a deep supervised self-encoder classification network based on multi-view feature learning, input multi-view morphological features to the encoder module, and then obtain high-order potential expressions of multi-view features , and then input the potential expression into the en...
Embodiment 3
[0133] This embodiment discloses an electronic device, including a memory and a processor, where a computer program is stored in the memory, and when the computer program is executed by the processor, the processor implements the method described in Embodiment 1 .
PUM
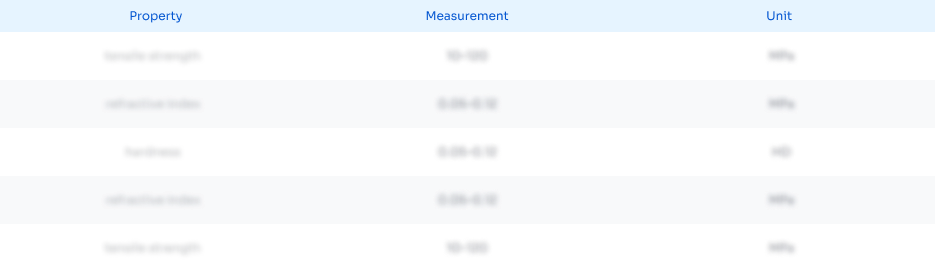
Abstract
Description
Claims
Application Information
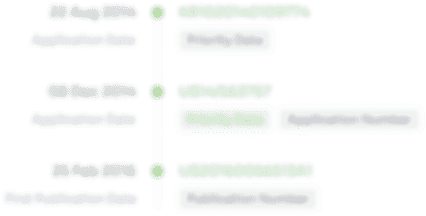
- R&D Engineer
- R&D Manager
- IP Professional
- Industry Leading Data Capabilities
- Powerful AI technology
- Patent DNA Extraction
Browse by: Latest US Patents, China's latest patents, Technical Efficacy Thesaurus, Application Domain, Technology Topic, Popular Technical Reports.
© 2024 PatSnap. All rights reserved.Legal|Privacy policy|Modern Slavery Act Transparency Statement|Sitemap|About US| Contact US: help@patsnap.com