Traffic congestion propagation prediction method based on space-time diagram convolutional neural network
A convolutional neural network and propagation prediction technology, applied in the field of traffic congestion propagation prediction based on spatiotemporal graph convolutional neural network, can solve the problems of poor prediction performance of congestion propagation model, complex traffic network, poor performance of prediction model, etc. , to achieve the effect of preventing and clearing traffic congestion
- Summary
- Abstract
- Description
- Claims
- Application Information
AI Technical Summary
Problems solved by technology
Method used
Image
Examples
Embodiment Construction
[0050] The specific embodiments of the present invention will be described in further detail below with reference to the accompanying drawings and embodiments. The following examples are intended to illustrate the present invention, but not to limit the scope of the present invention.
[0051] A traffic congestion propagation prediction method based on spatiotemporal graph convolutional neural network, such as figure 1 shown, including the following steps:
[0052] Step 1: Obtain traffic data source data, and make traffic speed dataset and sensor network adjacency matrix data;
[0053] Step 1.1: Download a state performance evaluation system (PeMS) traffic data set from the Internet; the traffic data set includes the location information of the speed sensor on the traffic road network and the traffic speed data detected by the sensor; the traffic speed data is divided into training datasets and validation datasets;
[0054] In this embodiment, the selected location for the ...
PUM
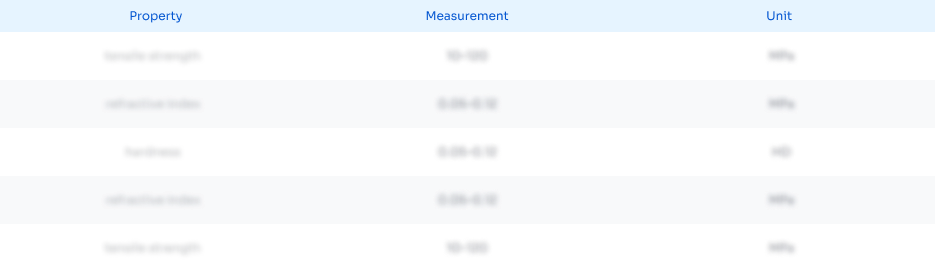
Abstract
Description
Claims
Application Information
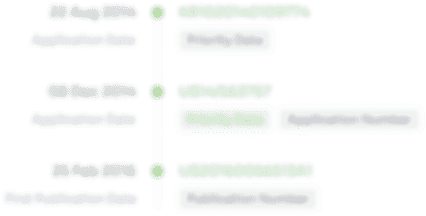
- Generate Ideas
- Intellectual Property
- Life Sciences
- Materials
- Tech Scout
- Unparalleled Data Quality
- Higher Quality Content
- 60% Fewer Hallucinations
Browse by: Latest US Patents, China's latest patents, Technical Efficacy Thesaurus, Application Domain, Technology Topic, Popular Technical Reports.
© 2025 PatSnap. All rights reserved.Legal|Privacy policy|Modern Slavery Act Transparency Statement|Sitemap|About US| Contact US: help@patsnap.com