Building surface crack detection method based on deep learning network
A technology of deep learning network and surface cracks, applied in neural learning methods, biological neural network models, image analysis, etc., can solve problems such as difficult to obtain crack shape, length and width, crack detection network model is not superior enough, etc., to simplify crack detection Process, model complexity is not high, the effect of high detection efficiency
- Summary
- Abstract
- Description
- Claims
- Application Information
AI Technical Summary
Problems solved by technology
Method used
Image
Examples
Embodiment
[0052] A method for detecting cracks on the surface of buildings based on deep learning network, the specific process is as follows figure 1 shown, including the following steps:
[0053] S1. Create an image training set.
[0054] The specific operation of the step S1 is as follows:
[0055] Step 1. Collect and augment the original data to form a data set containing 2000 crack images. The image is denoted as I, and the width and height are denoted as (W, H). The specific values are determined by the resolution of the acquisition equipment, such as ( W, H) can be (1280, 960);
[0056] Step 2, preprocessing the image, mainly including filtering and contrast enhancement, the filter kernel size is 3 × 3, using histogram equalization to carry out contrast enhancement on the image, and marking the preprocessed image as I';
[0057] Step 3. Use the Labelme software to mark I', the crack area is marked in green, and the non-crack area is marked in red, and the image is saved.
[0...
PUM
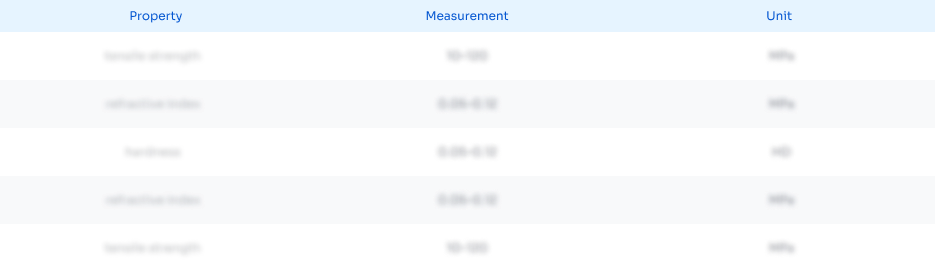
Abstract
Description
Claims
Application Information
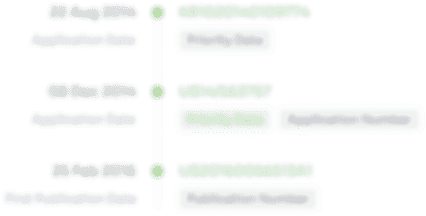
- R&D Engineer
- R&D Manager
- IP Professional
- Industry Leading Data Capabilities
- Powerful AI technology
- Patent DNA Extraction
Browse by: Latest US Patents, China's latest patents, Technical Efficacy Thesaurus, Application Domain, Technology Topic, Popular Technical Reports.
© 2024 PatSnap. All rights reserved.Legal|Privacy policy|Modern Slavery Act Transparency Statement|Sitemap|About US| Contact US: help@patsnap.com