Convolutional neural network hardware accelerator for solidifying full network layer on reconfigurable platform
A convolutional neural network and hardware accelerator technology, applied in the field of convolutional neural network hardware acceleration, can solve problems such as reduced computing performance, insufficient on-chip computing power, unbalanced utilization of off-chip memory access bandwidth, etc., to alleviate parallel computing The effect of conflicting resources
- Summary
- Abstract
- Description
- Claims
- Application Information
AI Technical Summary
Problems solved by technology
Method used
Image
Examples
Embodiment
[0060] The invention is oriented to efficient hardware deployment of convolutional neural networks, combines reconfigurable computing technology with heterogeneous multi-core architecture, and systematically proposes a heterogeneous multi-core accelerator structure and acceleration method on a reconfigurable platform, effectively alleviating Hardware-Software Feature Mismatch in Hardware Acceleration of Convolutional Neural Networks. In the present invention, a heterogeneous multi-core accelerator deployment method that solidifies the entire network layer on-chip is proposed. By realizing the end-to-end mapping between hierarchical computing and hardware structure, the adaptability between software and hardware features is improved, and traditional volume The waste of a large number of hardware resources in the design of the Accumulative Neural Network Accelerator improves the utilization efficiency of computing resources.
[0061] The overall architecture of the on-chip solid...
PUM
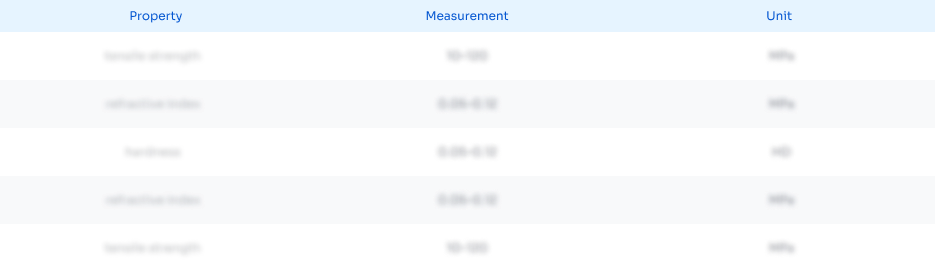
Abstract
Description
Claims
Application Information
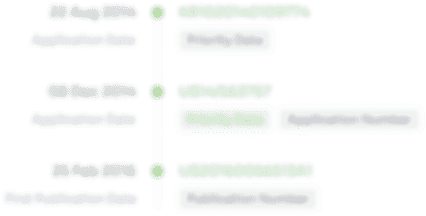
- Generate Ideas
- Intellectual Property
- Life Sciences
- Materials
- Tech Scout
- Unparalleled Data Quality
- Higher Quality Content
- 60% Fewer Hallucinations
Browse by: Latest US Patents, China's latest patents, Technical Efficacy Thesaurus, Application Domain, Technology Topic, Popular Technical Reports.
© 2025 PatSnap. All rights reserved.Legal|Privacy policy|Modern Slavery Act Transparency Statement|Sitemap|About US| Contact US: help@patsnap.com