Domain adaptive Faster R-CNN (Recurrent Convolutional Neural Network) semi-supervised SAR (Synthetic Aperture Radar) detection method
A semi-supervised and target detection technology, applied in the direction of neural learning methods, instruments, biological neural network models, etc., can solve the problems of test data SAR target detection performance degradation, Faster R-CNN detection performance degradation, etc., to achieve rich data, improve Detection performance, the effect of rich target information
- Summary
- Abstract
- Description
- Claims
- Application Information
AI Technical Summary
Problems solved by technology
Method used
Image
Examples
Embodiment 1
[0029] Most of the current SAR target detection methods require a large amount of labeled data for training, and these SAR target detection methods have a strong dependence on labeled samples. Because the number of SAR images is generally small, and marking requires a lot of manpower and material resources, it is difficult to obtain marked SAR images, and usually the data volume of marked SAR images is small. When there are few labeled SAR image training data, the features extracted by these SAR target detection methods have limited expression performance on the target, which further affects the detection performance, resulting in a sharp decline in the detection performance of the current CNN-based SAR target detection methods. Aiming at the above-mentioned status quo, the present invention conducts research and experiments, and proposes a semi-supervised SAR target detection method based on domain-adaptive Faster R-CNN, which can significantly improve the performance of SAR t...
Embodiment 2
[0050] The semi-supervised SAR target detection method based on domain adaptation Faster R-CNN is the same as embodiment 1, in the construction domain adaptation Faster R-CNN model described in the step (3) of the present invention, the basic network of the source domain and the basis of the target domain A constraint item based on the maximum mean difference (MMD) is added between the feature maps extracted by the networks to form an additional domain adaptation module. The expression of the constraint item based on the maximum mean difference (MMD) is as follows:
[0051] The data in the source domain and the target domain obtain corresponding feature maps through their respective basic networks, and the maximum mean difference (MMD) constraint is used between the feature maps of the source domain and target domain data, so that the i-th sample in the source domain and the kth sample of the target domain The corresponding feature map is expressed as and Then the featur...
Embodiment 3
[0057] The semi-supervised SAR target detection method based on domain adaptation Faster R-CNN is the same as embodiment 1-2, in the domain adaptation Faster R-CNN model constructed as described in step (3), the decoder structure connected after the basic network of the target domain It constitutes an additional decoder module, which is used to reconstruct the target domain data. The reconstruction is expressed as a constraint item, as follows:
[0058] The target domain data is adapted to the target domain basic network in Faster R-CNN to extract the feature map of the target domain data, and then the feature map of the target domain data is input into the decoder module, and the output of the decoder module is the input target domain data The reconstruction of , let the decoder for the k-th sample in the target domain data is refactored to Then the reconstructed constraints L recon Expressed as:
[0059]
[0060] Where||·|| 2 Indicates the L2 norm, N t Indicates th...
PUM
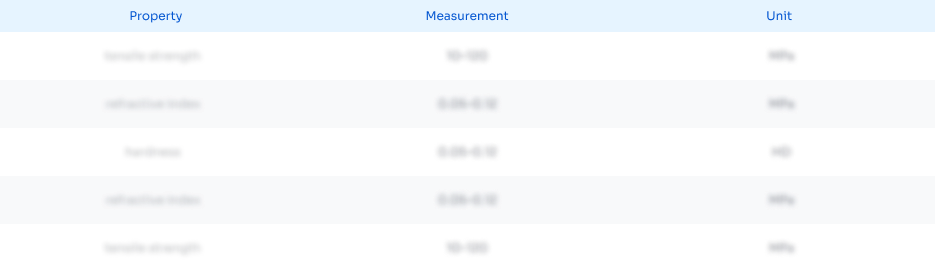
Abstract
Description
Claims
Application Information
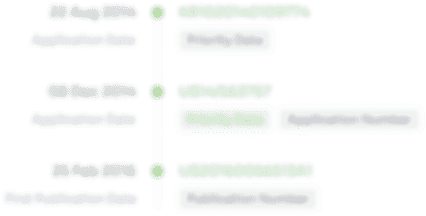
- R&D Engineer
- R&D Manager
- IP Professional
- Industry Leading Data Capabilities
- Powerful AI technology
- Patent DNA Extraction
Browse by: Latest US Patents, China's latest patents, Technical Efficacy Thesaurus, Application Domain, Technology Topic, Popular Technical Reports.
© 2024 PatSnap. All rights reserved.Legal|Privacy policy|Modern Slavery Act Transparency Statement|Sitemap|About US| Contact US: help@patsnap.com