A two-way prediction method of material microscopic image and performance based on deep learning
A microscopic image and deep learning technology, applied in neural learning methods, instruments, biological neural network models, etc., can solve the problems of high dissipation of plastic deformation, multiple types, and large scale span of microstructures, and achieve a good degree of fitting The effect of improving, improving accuracy, and avoiding model underfitting
- Summary
- Abstract
- Description
- Claims
- Application Information
AI Technical Summary
Problems solved by technology
Method used
Image
Examples
Embodiment Construction
[0047] The present invention will now be further described in conjunction with the embodiments and accompanying drawings:
[0048] The features contained in the material microscopic image of the present invention cannot meet the task of performance prediction, and further data mining is required to improve the feature engineering of the project. Considering that not only the material microstructure, but also the material composition, molding process and other factors affect the performance, other influencing factors can be synthesized into a one-dimensional feature vector.
[0049] The method for bidirectional prediction of material microscopic images and properties based on deep learning includes the following steps:
[0050] Step 1: Build a convolutional neural network to extract microscopic image features. The convolutional neural network uses the material image as the input of the network, which avoids the complex feature extraction and data reconstruction process in the ...
PUM
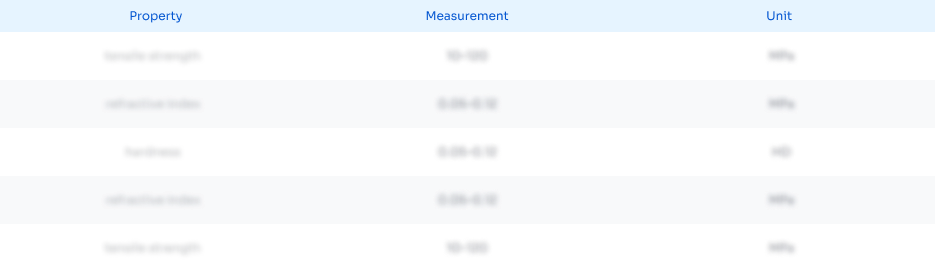
Abstract
Description
Claims
Application Information
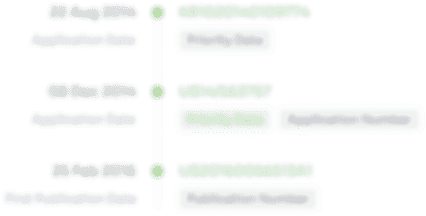
- R&D Engineer
- R&D Manager
- IP Professional
- Industry Leading Data Capabilities
- Powerful AI technology
- Patent DNA Extraction
Browse by: Latest US Patents, China's latest patents, Technical Efficacy Thesaurus, Application Domain, Technology Topic, Popular Technical Reports.
© 2024 PatSnap. All rights reserved.Legal|Privacy policy|Modern Slavery Act Transparency Statement|Sitemap|About US| Contact US: help@patsnap.com