Traffic scene risk assessment method and system based on multi-branch convolutional neural network
A convolutional neural network and traffic scene technology, applied in the field of traffic scene risk assessment based on multi-branch convolutional neural network, can solve the problems of inability to provide real-time and effective assessment results, inability to effectively assess road risks, and complex extraction model design process, etc. problem, to achieve the effect of improving information redundancy, improving accuracy, and solving overfitting
- Summary
- Abstract
- Description
- Claims
- Application Information
AI Technical Summary
Problems solved by technology
Method used
Image
Examples
Embodiment 1
[0047] The purpose of this embodiment is to provide a traffic scene risk assessment method based on a multi-branch convolutional neural network.
[0048] A traffic scene risk assessment method based on a multi-branch convolutional neural network, comprising:
[0049] Acquiring the traffic scene video, intercepting the video frame sequence according to the preset frequency, and calculating the optical flow graph between adjacent frames, and dividing the video frame sequence and the optical flow graph data into training set and test set respectively;
[0050] Construct a multi-branch convolutional neural network model, and embed a time-shift module and an attention module as a spatial branch network and a temporal branch network respectively;
[0051] Using the training set to train the multi-branch convolutional neural network model;
[0052] The test set is input into the trained multi-branch convolutional neural network model to generate evaluation scores of risk occurrence ...
Embodiment 2
[0156] The purpose of this embodiment is to provide a traffic scene risk assessment system based on a multi-branch convolutional neural network.
[0157] A traffic scene risk assessment system based on multi-branch convolutional neural network, including:
[0158] Data set construction module: obtain traffic scene video, intercept video frame sequence according to preset frequency, and calculate the optical flow diagram between adjacent frames, and divide the video frame sequence and optical flow diagram data into training set and test set respectively;
[0159] Model building module: build a multi-branch convolutional neural network model, and embed a time-shift module and an attention module as a spatial branch network and a time branch network respectively;
[0160] Model training module: using the training set to train the multi-branch convolutional neural network model;
[0161] Risk assessment module: input the test set into the trained multi-branch convolutional neural...
Embodiment 3
[0163] The purpose of this embodiment is to provide an electronic device.
[0164] An electronic device, including an image acquisition device, a memory, a processor, and a computer program stored on the memory, and the processor implements the above-mentioned multi-branch convolutional neural network-based traffic scene risk assessment when executing the program methods, including:
[0165] Acquiring the traffic scene video, intercepting the video frame sequence according to the preset frequency, and calculating the optical flow graph between adjacent frames, and dividing the video frame sequence and the optical flow graph data into training set and test set respectively;
[0166] Construct a multi-branch convolutional neural network model, and embed a time-shift module and an attention module as a spatial branch network and a temporal branch network respectively;
[0167] Using the training set to train the multi-branch convolutional neural network model;
[0168] The test...
PUM
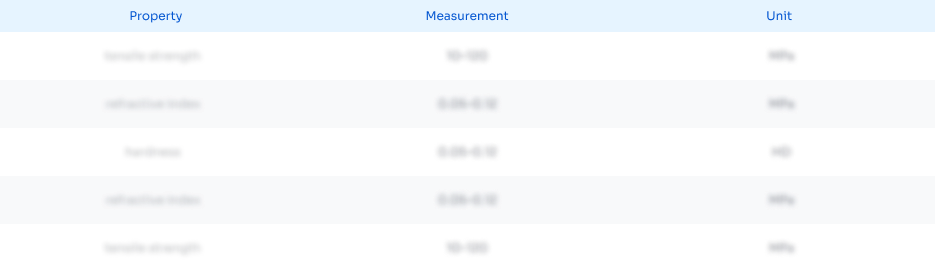
Abstract
Description
Claims
Application Information
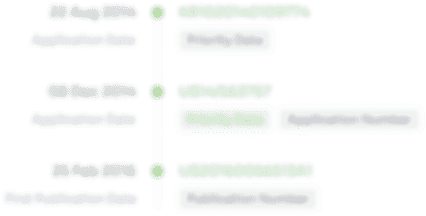
- R&D Engineer
- R&D Manager
- IP Professional
- Industry Leading Data Capabilities
- Powerful AI technology
- Patent DNA Extraction
Browse by: Latest US Patents, China's latest patents, Technical Efficacy Thesaurus, Application Domain, Technology Topic, Popular Technical Reports.
© 2024 PatSnap. All rights reserved.Legal|Privacy policy|Modern Slavery Act Transparency Statement|Sitemap|About US| Contact US: help@patsnap.com