Weakly supervised deep learning automatic bone age evaluation method
A deep learning and weakly supervised technology, applied in the field of medical artificial intelligence, can solve problems such as slow running speed, bloated model, and difficulty in quantifying personal experience, and achieve the effect of fast computing speed and small model
- Summary
- Abstract
- Description
- Claims
- Application Information
AI Technical Summary
Problems solved by technology
Method used
Image
Examples
Embodiment Construction
[0015] refer to figure 1
[0016] A weakly supervised deep learning automatic bone age assessment method, comprising the following steps:
[0017] Step 1: Data collection and data preprocessing
[0018] The present invention aims at bone age estimation of human beings, by taking X-ray films of the left hand, and predicting the bone age through the X-ray films. X-rays should include the entire hand and the radius. After the shooting, ask the expert doctor to mark the location of the key points. Before the X-ray film is processed by the model, it is uniformly transformed into a 512×512 size picture.
[0019] Step 2: Gesture detection and hand key point detection
[0020] Use Faster R-CNN as a gesture detection method for hand detection and hand key point detection. Among them, Faster R-CNN uses ResNet50 as the basic model, pre-trains on the COCO dataset, and then trains on the data of hand bone key point prediction. During the training process, the L1 loss function is use...
PUM
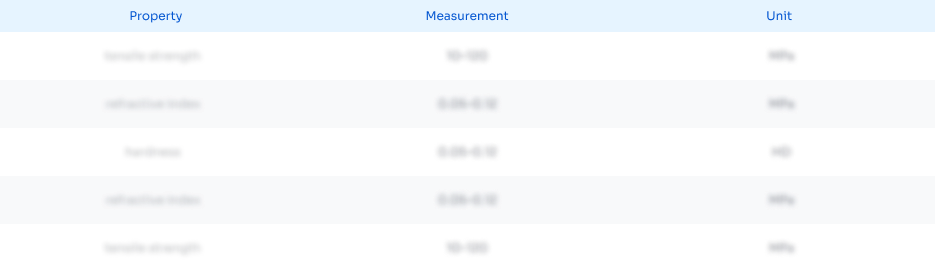
Abstract
Description
Claims
Application Information
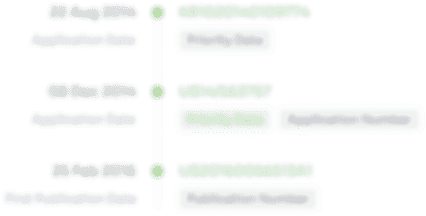
- R&D Engineer
- R&D Manager
- IP Professional
- Industry Leading Data Capabilities
- Powerful AI technology
- Patent DNA Extraction
Browse by: Latest US Patents, China's latest patents, Technical Efficacy Thesaurus, Application Domain, Technology Topic, Popular Technical Reports.
© 2024 PatSnap. All rights reserved.Legal|Privacy policy|Modern Slavery Act Transparency Statement|Sitemap|About US| Contact US: help@patsnap.com