Fractional order KVFD multi-parameter machine learning optimization method for viscoelasticity mechanical characterization of soft substance
A technology of elasticity and machine learning, applied in machine learning, design optimization/simulation, instruments, etc., can solve problems such as low efficiency, time-consuming and labor-intensive, low accuracy, and achieve good accuracy, improve accuracy and efficiency, Robust effect
- Summary
- Abstract
- Description
- Claims
- Application Information
AI Technical Summary
Problems solved by technology
Method used
Image
Examples
Embodiment Construction
[0064] In order to make the purpose, technical effects and technical solutions of the embodiments of the present invention more clear, the technical solutions in the embodiments of the present invention are clearly and completely described below in conjunction with the accompanying drawings in the embodiments of the present invention; obviously, the described embodiments It is a part of the embodiment of the present invention.
[0065] see figure 1 , a fractional-order KVFD multi-parameter machine learning optimization method for soft matter viscoelastic mechanical characterization according to an embodiment of the present invention is a method for automatically fitting KVFD model parameters according to corresponding curves, which can greatly improve KVFD model parameter fitting Speed and accuracy of parameters; it specifically includes the following steps:
[0066] Step 1. According to the solutions (relaxation, creep, load-unload) of the three different loading methods o...
PUM
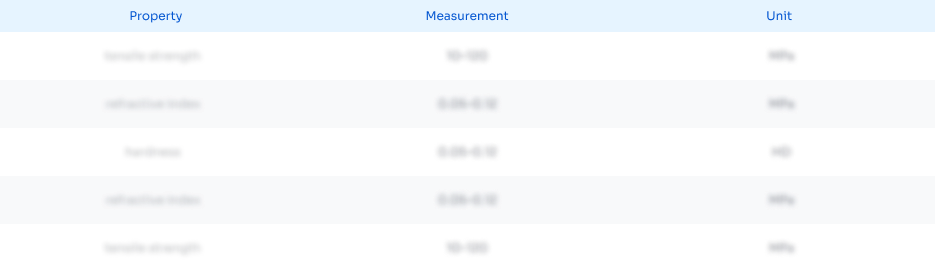
Abstract
Description
Claims
Application Information
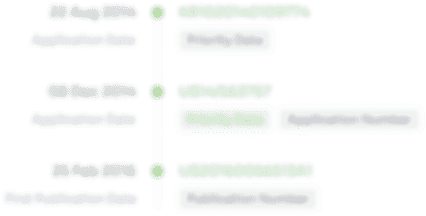
- R&D Engineer
- R&D Manager
- IP Professional
- Industry Leading Data Capabilities
- Powerful AI technology
- Patent DNA Extraction
Browse by: Latest US Patents, China's latest patents, Technical Efficacy Thesaurus, Application Domain, Technology Topic, Popular Technical Reports.
© 2024 PatSnap. All rights reserved.Legal|Privacy policy|Modern Slavery Act Transparency Statement|Sitemap|About US| Contact US: help@patsnap.com