Three-branch convolutional network fabric defect detection method based on weak supervised learning
A convolutional network and detection method technology, applied in neural learning methods, biological neural network models, image analysis, etc., can solve problems such as missing labels in datasets, achieve enhanced useful features, improve detection accuracy and adaptability, and avoid interference effect
- Summary
- Abstract
- Description
- Claims
- Application Information
AI Technical Summary
Problems solved by technology
Method used
Image
Examples
specific example
[0080]In the embodiment, the present invention randomly selects 3000 images containing defects from the image library of the fabric production industrial site, and the image size is selected as 512pixel×512pixel. During training and testing, the learning rate is set to 1e-5, the momentum parameter is set to 0.2, and the weight decay is set to 0.0005. The fusion weights in the feature fusion module are all initialized to a normal distribution during the training phase. For specific examples, see Figure 5-Figure 10 .
[0081] Figure 5 (a)~(d) are original defect pictures; Figure 6 (a)~(d) are documents [1]-[Schlemper J, Oktay O, Chen L, et al. Attention-Gated Networks for Improving Ultrasound Scan PlaneDetection.[J].arXiv:Computer Vision and Pattern Recognition,2018. ] method generated heat map (generated by the weighted combination between the defect image and the corresponding class activation map); Figure 7 (a)~(d) are the class activation maps generated by [1]. Thi...
PUM
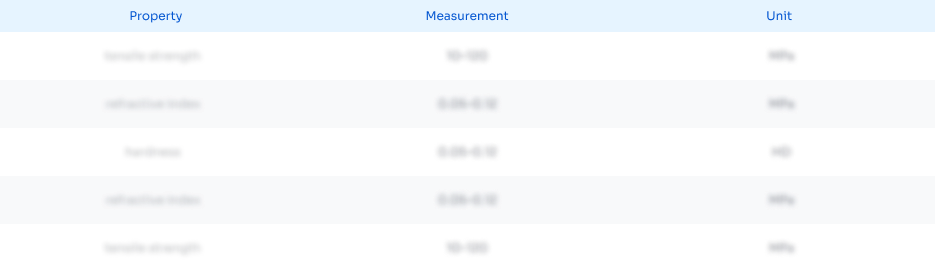
Abstract
Description
Claims
Application Information
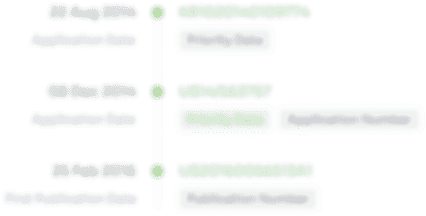
- R&D Engineer
- R&D Manager
- IP Professional
- Industry Leading Data Capabilities
- Powerful AI technology
- Patent DNA Extraction
Browse by: Latest US Patents, China's latest patents, Technical Efficacy Thesaurus, Application Domain, Technology Topic.
© 2024 PatSnap. All rights reserved.Legal|Privacy policy|Modern Slavery Act Transparency Statement|Sitemap