Multi-modal small sample learning method based on significance
A learning method and multi-modal technology, applied in character and pattern recognition, biological neural network models, instruments, etc., can solve problems such as costly manpower and financial resources, limited model applicability, data difficulties, etc., to enhance classification ability, enrich Feature representation, the effect of enhancing usability
- Summary
- Abstract
- Description
- Claims
- Application Information
AI Technical Summary
Problems solved by technology
Method used
Image
Examples
Embodiment Construction
[0023] The present invention will be described in further detail below in conjunction with the accompanying drawings and specific embodiments. It should be understood that the specific embodiments described here are only used to explain the present invention, not to limit the present invention.
[0024] The saliency-based multimodal small sample learning method provided by the present invention mainly adds saliency image extraction, multimodal information combination and label propagation operations on the basis of traditional small sample classification. First, the saliency map of the image is obtained through the saliency detection network to obtain the foreground and background regions of the image, and then the semantic information and the visual information are combined through the multi-modal hybrid model, and the semantic information is used to assist the visual information to classify, and finally, the data is used The manifold structure of the data sample is construct...
PUM
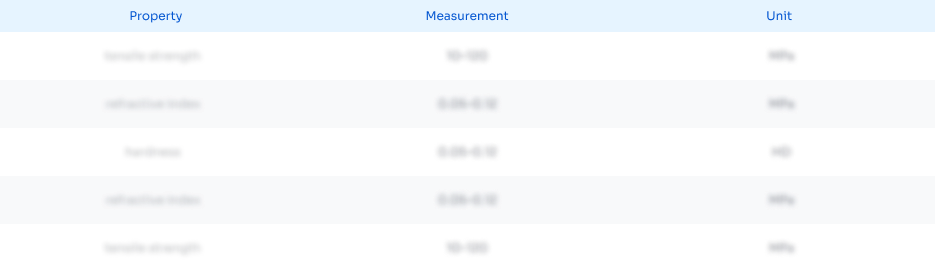
Abstract
Description
Claims
Application Information
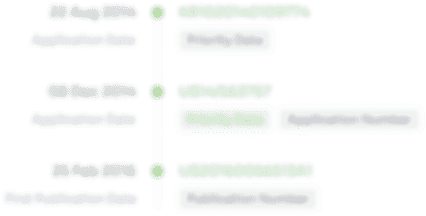
- R&D Engineer
- R&D Manager
- IP Professional
- Industry Leading Data Capabilities
- Powerful AI technology
- Patent DNA Extraction
Browse by: Latest US Patents, China's latest patents, Technical Efficacy Thesaurus, Application Domain, Technology Topic, Popular Technical Reports.
© 2024 PatSnap. All rights reserved.Legal|Privacy policy|Modern Slavery Act Transparency Statement|Sitemap|About US| Contact US: help@patsnap.com