A method for screening disease-associated proteins based on complex networks
A technology for complex networks and related proteins, applied in the field of screening disease-related proteins based on complex networks, can solve the problems of under-fitting, over-fitting, and insufficiency of models, and achieve the effects of improving accuracy, reducing workload, and improving accuracy.
- Summary
- Abstract
- Description
- Claims
- Application Information
AI Technical Summary
Problems solved by technology
Method used
Image
Examples
Embodiment 1
[0038] Example 1: Coronary heart disease
[0039] This embodiment shows the technical effect 1 of the invention. Using the node2vec algorithm to extract the characteristic structure of the protein in the protein interaction network, compared with the traditional topological properties, it can improve the accuracy of protein recognition in the protein interaction network. In the S5 part of this example, the combined algorithm of "node2vec algorithm and PU-learning algorithm" was used to obtain 958 coronary heart disease-related proteins, with an accuracy rate (92.35%) and a recall rate (56.89%); while using the "topological property algorithm and PU-learning algorithm" "learning algorithm" algorithm combination, and obtained 8348 proteins related to coronary heart disease, with a precision rate (32.93%) and a recall rate (10.66%).
[0040] Such as figure 1 As shown, the embodiment of the present invention provides a method for screening disease-related proteins based on a comp...
Embodiment 2
[0046] Embodiment two: ischemic cardiomyopathy
[0047] In this embodiment, in order to protect the parameter range and dimension d of the method node2vec, a dimension of 128-256 is selected to obtain a more stable protein interaction network analysis result. When the dimension is 64, the RN results of the stable negative sample set are less. When the dimension is 512, the sensitivity of the data model on the verification set is greatly reduced.
[0048] S1: Obtain 270 seed genes related to ischemic cardiomyopathy based on GWAS catalog, IPA, DisGeNET and other databases (set P);
[0049] S2: Based on the protein-protein interaction database (BIOGRID, HPRD, INTACT, STRING protein interaction database), construct a protein interaction network with the seed gene of ischemic cardiomyopathy as the core, the network consists of 9329 proteins, 30274 protein- The composition of protein interaction relationship; 263 of the 270 seed genes in S1 were identified in the protein interactio...
Embodiment 3
[0054] Example Three: Atrial Fibrillation
[0055] In this embodiment, in order to protect the parameter range of the method node2vec, the preferred range of p is [2, 5], and the preferred range of q is [0.1, 3].
[0056] S1: Based on GWAS catalog, Malacards, DisGeNET and other databases, 141 atrial fibrillation-related seed genes (set P) were obtained;
[0057] S2: Based on the protein-protein interaction database (BIOGRID, HPRD, INTACT, STRING protein interaction database), construct a protein interaction network with atrial fibrillation seed gene as the core, which consists of 5745 proteins and 13606 protein-protein interactions Relationship composition; 131 of the 141 seed genes in S1 were identified in the protein interaction database, and 10 proteins were not identified.
[0058] S3: Based on the node2vec algorithm, extract the characteristic data of 9329 proteins in the S2 protein interaction network; during the implementation process, set the random walk parameters wa...
PUM
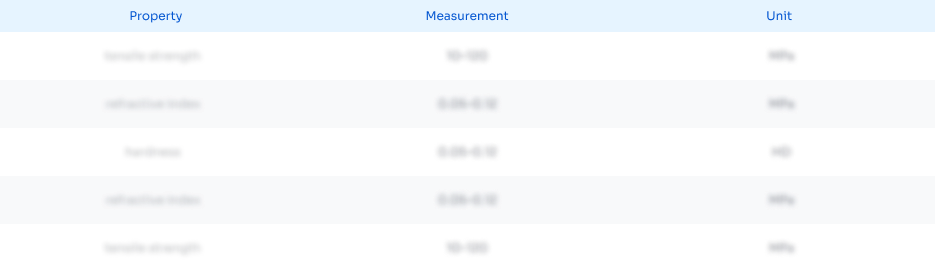
Abstract
Description
Claims
Application Information
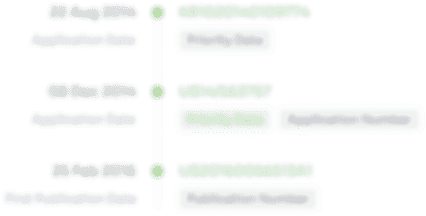
- R&D Engineer
- R&D Manager
- IP Professional
- Industry Leading Data Capabilities
- Powerful AI technology
- Patent DNA Extraction
Browse by: Latest US Patents, China's latest patents, Technical Efficacy Thesaurus, Application Domain, Technology Topic, Popular Technical Reports.
© 2024 PatSnap. All rights reserved.Legal|Privacy policy|Modern Slavery Act Transparency Statement|Sitemap|About US| Contact US: help@patsnap.com