Defective workpiece image recognition method based on convolutional attention neural network
A convolutional neural network and neural network technology, applied in the field of defect workpiece image recognition based on convolutional attention neural network, can solve the problems of small defects being easily ignored and low recognition rate, so as to improve the accuracy rate and expand the data set , a wide range of effects
- Summary
- Abstract
- Description
- Claims
- Application Information
AI Technical Summary
Problems solved by technology
Method used
Image
Examples
Embodiment Construction
[0021] The present invention will be further described below in conjunction with embodiment, should be understood that these embodiments are only used to illustrate the present invention and are not intended to limit the scope of the present invention, after having read the present invention, those skilled in the art can modify various equivalent forms of the present invention All fall within the scope defined by the appended claims of this application.
[0022] Please combine figure 1 As shown, the present embodiment relates to a defect workpiece image recognition method based on a convolutional attention neural network, including
[0023] Feature extraction: Based on the network structure of the convolutional neural network, the feature extraction network is formed after pre-training the weights on the dataset ImageNet, and the image X∈R (H×W) , input into the feature extraction network to obtain the corresponding deep features;
[0024] The network structure of the convol...
PUM
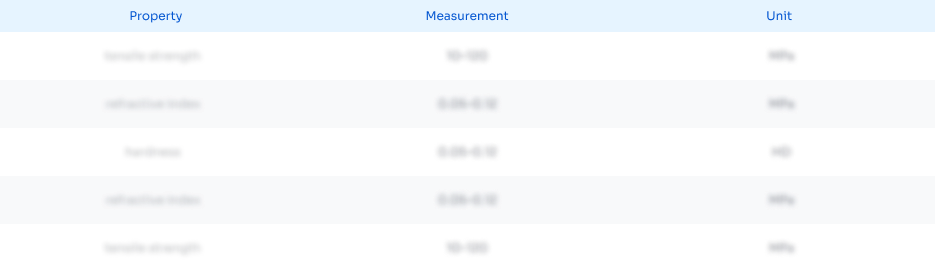
Abstract
Description
Claims
Application Information
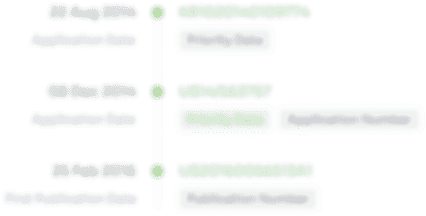
- Generate Ideas
- Intellectual Property
- Life Sciences
- Materials
- Tech Scout
- Unparalleled Data Quality
- Higher Quality Content
- 60% Fewer Hallucinations
Browse by: Latest US Patents, China's latest patents, Technical Efficacy Thesaurus, Application Domain, Technology Topic, Popular Technical Reports.
© 2025 PatSnap. All rights reserved.Legal|Privacy policy|Modern Slavery Act Transparency Statement|Sitemap|About US| Contact US: help@patsnap.com