An auxiliary diagnosis system for Alzheimer's disease based on fnirs and graph neural network
A technology of Alzheimer's disease and neural network, which is applied in the field of auxiliary diagnosis system for Alzheimer's disease, can solve the problems of different time domain and frequency domain of EEG signals, and achieve comprehensive descriptiveness, good accuracy and effectiveness Effect
Active Publication Date: 2021-08-03
SHANDONG UNIV
View PDF9 Cites 0 Cited by
- Summary
- Abstract
- Description
- Claims
- Application Information
AI Technical Summary
Problems solved by technology
The inventors found that patients with cognitive impairment have differences in physiological signals such as EEG signals and normal people, and the parameters of EEG signals in the time domain and frequency domain are different from those of normal people; at present, there is no use of fNIRS data as Based on the input of the GCN network, and according to the fact that patients with cognitive impairment have differences in physiological signals such as EEG signals and normal people, a scheme for auxiliary diagnosis of Alzheimer's disease is proposed.
Method used
the structure of the environmentally friendly knitted fabric provided by the present invention; figure 2 Flow chart of the yarn wrapping machine for environmentally friendly knitted fabrics and storage devices; image 3 Is the parameter map of the yarn covering machine
View moreImage
Smart Image Click on the blue labels to locate them in the text.
Smart ImageViewing Examples
Examples
Experimental program
Comparison scheme
Effect test
Embodiment 1
[0043] Such as figure 1 As shown, the present embodiment provides an auxiliary diagnosis system for Alzheimer's disease based on fNIRS and graph neural network, including:
the structure of the environmentally friendly knitted fabric provided by the present invention; figure 2 Flow chart of the yarn wrapping machine for environmentally friendly knitted fabrics and storage devices; image 3 Is the parameter map of the yarn covering machine
Login to view more PUM
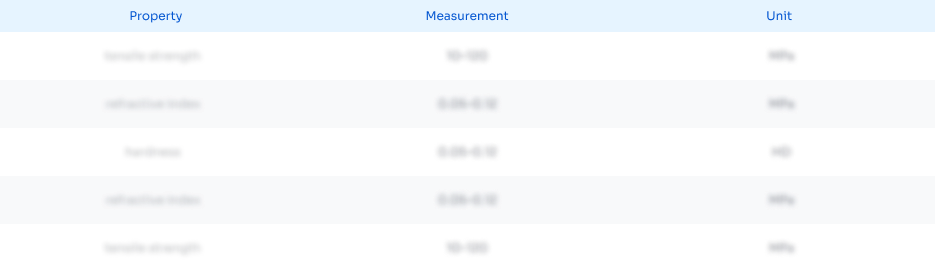
Abstract
The disclosure discloses an auxiliary diagnosis system for Alzheimer's disease based on fNIRS and a graph neural network, including an EEG detection module using a functional near-infrared spectrum imaging device to oxygenate the brains of AD patients and normal people in an active state Multi-channel detection of hemoglobin, deoxygenated hemoglobin and total hemoglobin, and construction of the fNIRS dataset; the adjacency matrix construction module uses the correlation between channels to construct the adjacency matrix for the fNIRS dataset; the node feature matrix construction module performs feature extraction on the fNIRS dataset, Construct the node feature matrix; the graph structure building module constructs the AD patient graph structure and the normal person graph structure respectively according to the adjacency matrix and the node feature matrix to form a training data set; the Alzheimer's disease recognition module constructs a graph neural network based on the training data set pair Carry out training, use the trained graph neural network to identify the test data set of the person to be tested, and output the auxiliary diagnosis result. Realize the use of graph neural networks in the classification of fNIRS data.
Description
technical field [0001] The present disclosure relates to the technical field of deep learning, in particular to an auxiliary diagnosis system for Alzheimer's disease based on fNIRS and a graph neural network. Background technique [0002] The statements in this section merely provide background information related to the present disclosure and do not necessarily constitute prior art. [0003] Graph Convolutional Neural Network (GCN) directly acts on the graph structure through a feature extractor, which is used to model the dependencies between graph nodes. Currently, most graph convolutional neural networks have a similar structure due to the use of convolution operators that can share weights across the graph. Compared with cumbersome fully-connected models, this neural network structure has at least three advantages in graph data: 1) it avoids the parameter explosion associated with fully-connected layers; 2) it allows parameters to be shared across the network and avoid...
Claims
the structure of the environmentally friendly knitted fabric provided by the present invention; figure 2 Flow chart of the yarn wrapping machine for environmentally friendly knitted fabrics and storage devices; image 3 Is the parameter map of the yarn covering machine
Login to view more Application Information
Patent Timeline
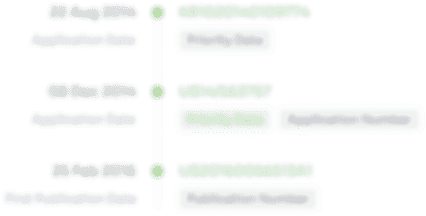
Patent Type & Authority Patents(China)
IPC IPC(8): A61B5/00
CPCA61B5/0042A61B5/0075A61B5/7267
Inventor 刘治杨燕芳孙健
Owner SHANDONG UNIV
Who we serve
- R&D Engineer
- R&D Manager
- IP Professional
Why Eureka
- Industry Leading Data Capabilities
- Powerful AI technology
- Patent DNA Extraction
Social media
Try Eureka
Browse by: Latest US Patents, China's latest patents, Technical Efficacy Thesaurus, Application Domain, Technology Topic.
© 2024 PatSnap. All rights reserved.Legal|Privacy policy|Modern Slavery Act Transparency Statement|Sitemap