Signal identification attack defense method based on generative adversarial network
A network attack, generative technology, applied in biological neural network models, wireless communication, electrical components, etc., can solve problems such as adversarial attacks, signal transmission security risks, security risks, etc.
- Summary
- Abstract
- Description
- Claims
- Application Information
AI Technical Summary
Problems solved by technology
Method used
Image
Examples
Embodiment Construction
[0055] The present invention will be further described below in conjunction with the accompanying drawings.
[0056] refer to Figure 1 ~ Figure 4 , a defense method based on generative against network attacks, including the following steps:
[0057] 1) Use the long short-term memory network (LSTM) to build a suitable generative confrontation network structure GAN.
[0058] Among them, the generative confrontation network structure built by LSTM, referred to as GAN. It includes a generative model G for outputting adversarial samples based on input benign samples and a discriminative model D for judging the authenticity of input adversarial samples. It is necessary to try to make the network complexity of the generative model and the discriminative model similar to each other, so as to ensure that the maximum effect of game training can be achieved as much as possible during the mutual training of the two models.
[0059] Because the generative adversarial network adopts the...
PUM
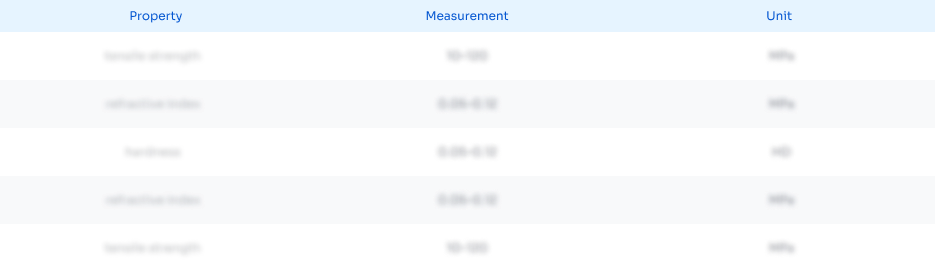
Abstract
Description
Claims
Application Information
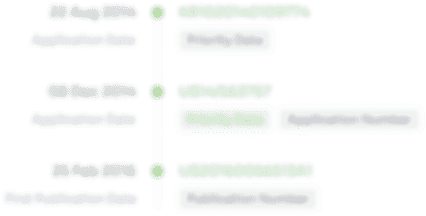
- R&D Engineer
- R&D Manager
- IP Professional
- Industry Leading Data Capabilities
- Powerful AI technology
- Patent DNA Extraction
Browse by: Latest US Patents, China's latest patents, Technical Efficacy Thesaurus, Application Domain, Technology Topic, Popular Technical Reports.
© 2024 PatSnap. All rights reserved.Legal|Privacy policy|Modern Slavery Act Transparency Statement|Sitemap|About US| Contact US: help@patsnap.com