A method for constructing quantum feedforward neural network method based on classic training
A feedforward neural network and training method technology, applied in neural learning methods, biological neural network models, neural architectures, etc., can solve problems such as the way that quantum neuron input is not considered, and the quantum neural network model has no training process. Good ductility
- Summary
- Abstract
- Description
- Claims
- Application Information
AI Technical Summary
Problems solved by technology
Method used
Image
Examples
Embodiment Construction
[0045] The present disclosure provides a method for constructing a quantum feedforward neural network based on classical training. The method for constructing a quantum feedforward neural network based on classical training provides a definition of a quantum neuron, which constitutes a quantum feedforward neural network. The internet. The input, output and weight of each neuron in the quantum feedforward neural network are all quantum states, and the implementation of the activation function of each neuron has a specific quantum circuit. The quantum feedforward neural network has scalability, and a quantum circuit scheme of any scale quantum feedforward neural network is given. The quantum feed-forward neural network is trained using a classical training method, and the effectiveness of classical training is quantitatively analyzed.
[0046] In order to make the purpose, technical solutions and advantages of the present disclosure clearer, the present disclosure will be furth...
PUM
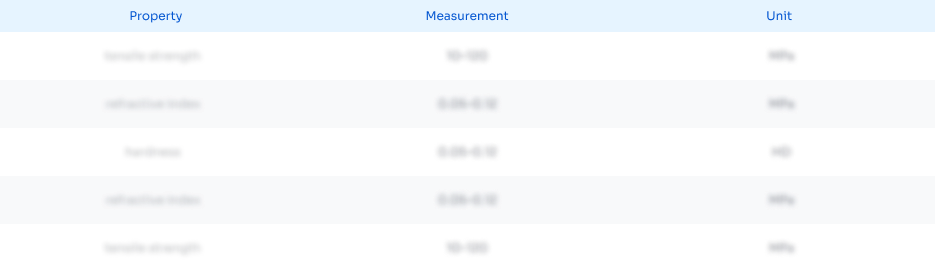
Abstract
Description
Claims
Application Information
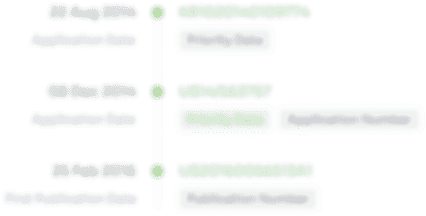
- R&D Engineer
- R&D Manager
- IP Professional
- Industry Leading Data Capabilities
- Powerful AI technology
- Patent DNA Extraction
Browse by: Latest US Patents, China's latest patents, Technical Efficacy Thesaurus, Application Domain, Technology Topic, Popular Technical Reports.
© 2024 PatSnap. All rights reserved.Legal|Privacy policy|Modern Slavery Act Transparency Statement|Sitemap|About US| Contact US: help@patsnap.com