Robust automatic face fusion method
An automatic face and fusion method technology, applied in the field of image synthesis, can solve the problems of poor fusion of image features, not natural enough, and no perfect solution to the occlusion problem, achieving the effect of wide applicability and expanding boundaries
- Summary
- Abstract
- Description
- Claims
- Application Information
AI Technical Summary
Problems solved by technology
Method used
Image
Examples
Embodiment 1
[0029] Please refer to figure 1 and figure 2 , the embodiment of the present invention provides a robust automatic face fusion method, comprising the following steps:
[0030] S1. Obtain two face images, which are face image A and face image B respectively;
[0031] S2. Perform occlusion processing on the face image A and the face image B respectively to obtain the four-channel image A and the four-channel image B. The four-channel image A contains the identity features in the composite image, and a non-occlusion mask is added relative to the image A. As a feature of a channel, the four-channel image B contains the attribute (non-identity) features in the composite image. Compared with the image B, a non-occlusion mask is added as a feature of a channel. The specific methods for occlusion processing of the face image include the following step:
[0032] S21. Use the available large batch of segmentation data to train an initial segmentation model, use the small batch of fa...
Embodiment 2
[0042] Please refer to image 3 , for step S3 of Embodiment 1, it uses the VGG network to encode the four-channel image A to obtain the encoding feature A, and uses the VAE encoder to encode the four-channel image B to obtain the encoding feature B.
[0043] In this embodiment, VGG is used for encoding identity features, which is a classic CNN network, and variational autoencoder VAE is used for encoding non-identity features. The reason for this is that identity features can be extracted under supervision. We clearly know the meaning that the extracted features should represent, which is the identity of a certain face, so we can use the feature extraction module in the pre-trained face recognition model to directly identify Extract facial features from images. For non-identity features, it may include picture background, lighting, face posture, expression, etc. We don’t even know what types of features need to be extracted, we only know that all features that have nothing to d...
Embodiment 3
[0047] For step S4 of Embodiment 1, the generative confrontation network includes a generator and a discriminator, wherein the generator is used to combine the encoding feature A and the encoding feature B to obtain a synthetic face image, and the discriminator is used to judge the face synthetic image authenticity.
[0048] In this embodiment, the discriminator is used to judge whether the image synthesized by the generator is real or not, and calculates the gap between the synthesized sample and the real sample through the loss function, which is called the current training loss of the sample. Then the network will use the optimization algorithm of gradient descent to adjust the network parameters according to the training loss, so that the training loss will be further reduced, that is, the degree of fake images will be further increased. The discriminator is only needed in the network model training phase, not in the application phase.
PUM
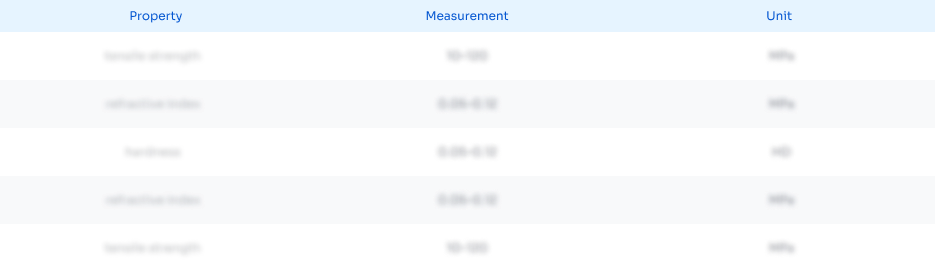
Abstract
Description
Claims
Application Information
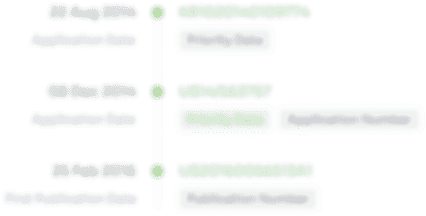
- R&D Engineer
- R&D Manager
- IP Professional
- Industry Leading Data Capabilities
- Powerful AI technology
- Patent DNA Extraction
Browse by: Latest US Patents, China's latest patents, Technical Efficacy Thesaurus, Application Domain, Technology Topic, Popular Technical Reports.
© 2024 PatSnap. All rights reserved.Legal|Privacy policy|Modern Slavery Act Transparency Statement|Sitemap|About US| Contact US: help@patsnap.com