Image dimension reduction method of extreme learning machine based on graph embedding
An extreme learning machine and graph embedding technology, which is applied in the field of image dimensionality reduction of extreme learning machines, can solve problems such as damage to the original sample distance relationship, insufficient stability of autoencoder performance, and neglect of label information, etc., to achieve improved class discrimination, Effects of Stability Guarantee and Strong Class Discrimination
- Summary
- Abstract
- Description
- Claims
- Application Information
AI Technical Summary
Problems solved by technology
Method used
Image
Examples
Embodiment Construction
[0049] The present invention proposes an image dimensionality reduction method for an extreme learning machine based on graph embedding. The present invention will be further described in detail below in conjunction with the accompanying drawings and specific embodiments.
[0050] The present invention proposes an image dimensionality reduction method (GDR-ELM) based on a graph embedding extreme learning machine, which includes four stages: initialization of an autoencoder, establishment of a graph embedding matrix, calculation of a feature extraction matrix and feature extraction. The technical solution of the present invention is as follows: firstly, construct a sample relationship matrix by using the distance between samples and label information of the original image samples; then according to the constructed sample relationship matrix, first perform random mapping on the input vectorized image, and then by minimizing Weighting the sample reconstruction error to learn the f...
PUM
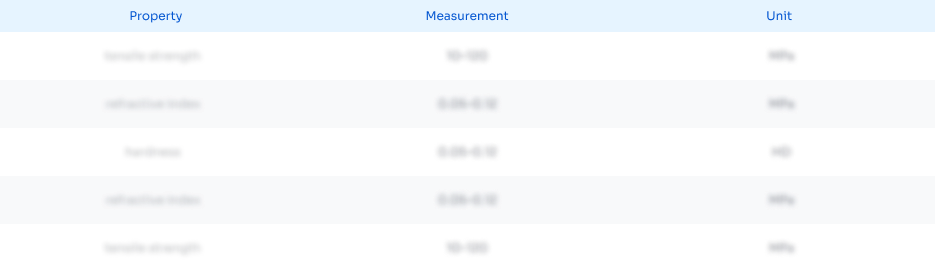
Abstract
Description
Claims
Application Information
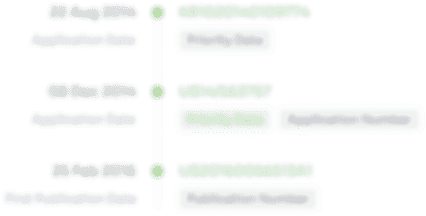
- R&D Engineer
- R&D Manager
- IP Professional
- Industry Leading Data Capabilities
- Powerful AI technology
- Patent DNA Extraction
Browse by: Latest US Patents, China's latest patents, Technical Efficacy Thesaurus, Application Domain, Technology Topic, Popular Technical Reports.
© 2024 PatSnap. All rights reserved.Legal|Privacy policy|Modern Slavery Act Transparency Statement|Sitemap|About US| Contact US: help@patsnap.com