Steel material design method based on physical-guided machine learning algorithm
A machine learning, steel material technology, applied in computer materials science, nuclear methods, instruments, etc., can solve problems such as reducing the research and development efficiency of PM models, limiting model optimization, complex microstructure characterization, etc., to improve model generalization ability, Design efficient effects
- Summary
- Abstract
- Description
- Claims
- Application Information
AI Technical Summary
Problems solved by technology
Method used
Image
Examples
Embodiment Construction
[0045] The specific implementation manners of the present invention will be further described in detail below in conjunction with the accompanying drawings and embodiments. The following examples are used to illustrate the present invention, but are not intended to limit the scope of the present invention.
[0046] In the present invention, the physical metallurgical parameters highly related to the target performance are added to the data set to participate in the model training and design process, making the machine learning process rich in physical meaning. At the same time, the genetic algorithm is used to optimize the design of the composition process, and finally the classifier is used to efficiently screen the design results. The design process is as follows figure 1 shown. Compared with pure machine learning design results, the prediction accuracy of machine learning under the guidance of physical metallurgy is higher, and the design results are more in line with the ...
PUM
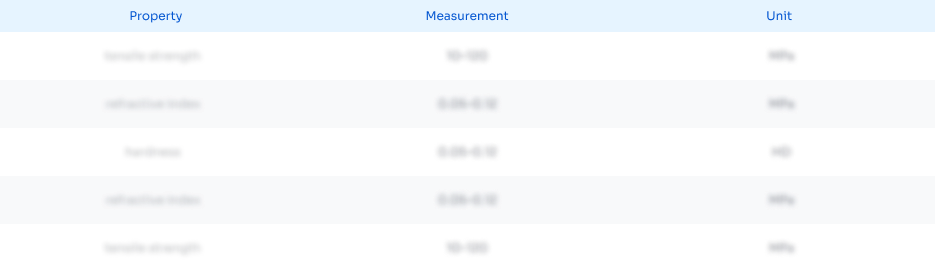
Abstract
Description
Claims
Application Information
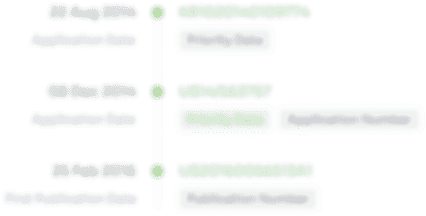
- R&D Engineer
- R&D Manager
- IP Professional
- Industry Leading Data Capabilities
- Powerful AI technology
- Patent DNA Extraction
Browse by: Latest US Patents, China's latest patents, Technical Efficacy Thesaurus, Application Domain, Technology Topic, Popular Technical Reports.
© 2024 PatSnap. All rights reserved.Legal|Privacy policy|Modern Slavery Act Transparency Statement|Sitemap|About US| Contact US: help@patsnap.com