Visual question and answer enhancement method based on graph convolution
A vision and convolution technology, applied in the fields of computer vision and natural language, can solve problems such as not being able to explore high-level semantics well, and achieve the effect of improving accuracy
- Summary
- Abstract
- Description
- Claims
- Application Information
AI Technical Summary
Problems solved by technology
Method used
Image
Examples
Embodiment Construction
[0014] In order to make the above objects, features and advantages of the present invention more comprehensible, the present invention will be further described in detail below in conjunction with the accompanying drawings and specific embodiments.
[0015] The visual question answering enhancement method based on graph convolution proposed by the present invention, such as figure 1 As shown, the first step of our model is to first extract features, use GRU to obtain the feature representation of the problem, and use the output of the bottom-up attention model extracted from Faster R-CNN as the feature representation of the image;
[0016] Then the graph learner learns the adjacency matrix of the image objects based on the question, and adds the relations between the objects detected by the relational feature detector. Finally we process graph features and combine them with questions to form a multi-class classification to predict the correct answer.
[0017] The specific imp...
PUM
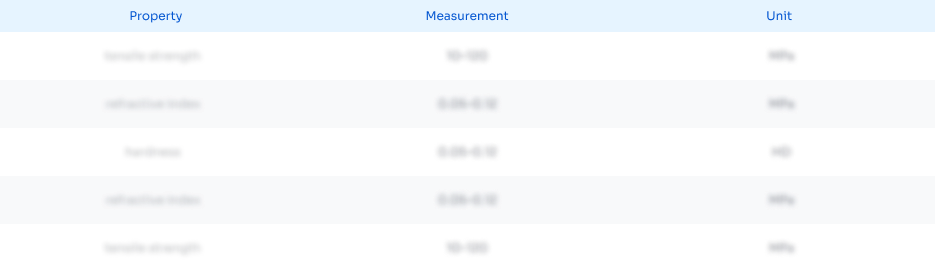
Abstract
Description
Claims
Application Information
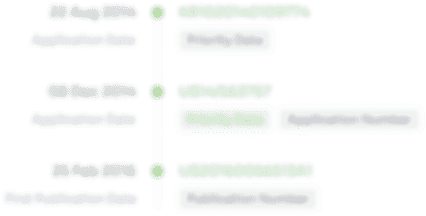
- R&D Engineer
- R&D Manager
- IP Professional
- Industry Leading Data Capabilities
- Powerful AI technology
- Patent DNA Extraction
Browse by: Latest US Patents, China's latest patents, Technical Efficacy Thesaurus, Application Domain, Technology Topic, Popular Technical Reports.
© 2024 PatSnap. All rights reserved.Legal|Privacy policy|Modern Slavery Act Transparency Statement|Sitemap|About US| Contact US: help@patsnap.com