Double-supervised image dehazing method, system, medium and equipment based on deep learning
A deep learning, dual-supervision technology, applied in the field of image processing, can solve problems such as affecting the dehazing effect, and achieve the effect of avoiding gradient explosion, avoiding gradient disappearance, and reducing training time.
- Summary
- Abstract
- Description
- Claims
- Application Information
AI Technical Summary
Problems solved by technology
Method used
Image
Examples
Embodiment 1
[0070] In this embodiment, a double-supervised lightweight image defogging system based on deep learning is provided, including: a foggy image and a fogless image sample acquisition module, a neural network system building module and a neural network system training module;
[0071] The foggy image and fog-free image sample acquisition module is used to acquire foggy image and fog-free image samples, and the fog-free image samples are used as label comparison samples;
[0072] Such as figure 1 As shown, the neural network system building block is used to construct the neural network system, and the neural network system includes a down-sampling module, a feature extraction module and an up-sampling module;
[0073] Downsampling module: The downsampling module includes a convolutional layer and a maximum pooling layer. The convolutional layer extracts image features, and then uses the maximum pooling operation. Its function is to reduce the size of the image, so that subsequent...
Embodiment 2
[0084] Such as figure 2 As shown, the present embodiment provides a double-supervised image defogging method based on deep learning, comprising the following steps:
[0085] S1: Obtain foggy images and tag non-foggy images;
[0086] S2: Build a neural network system, initialize the convolution kernel weights of the neural network to a Gaussian random distribution, and set the deviation value; in this embodiment, initialize the convolution kernel weight w in the neural network to a mean value of 0 and a variance of Gaussian random distribution of 0.05, the deviation b is set to a constant of 0.1;
[0087] The specific construction steps of the neural network system are as follows:
[0088] S21: Downsampling: Extract image features through the convolutional layer, extract the most significant features of the image through the maximum pooling layer, and reduce the image size, so that subsequent network calculations can be completed faster;
[0089] S22: Feature extraction: ad...
Embodiment 3
[0130] This embodiment also provides a storage medium, the storage medium may be a storage medium such as ROM, RAM, magnetic disk, optical disk, etc., and the storage medium stores one or more programs. When the programs are executed by the processor, the implementation of Embodiment 2 based A double-supervised image defogging method for deep learning, the method includes the following steps:
[0131] S1: Obtain foggy images and tag non-foggy images;
[0132] S2: Build a neural network system, initialize the convolution kernel weights of the neural network to a Gaussian random distribution, and set the deviation value; in this embodiment, initialize the convolution kernel weight w in the neural network to a mean value of 0 and a variance of Gaussian random distribution of 0.05, the deviation b is set to a constant of 0.1;
[0133] The specific construction steps of the neural network system are as follows:
[0134] S21: Downsampling: Extract image features through the convol...
PUM
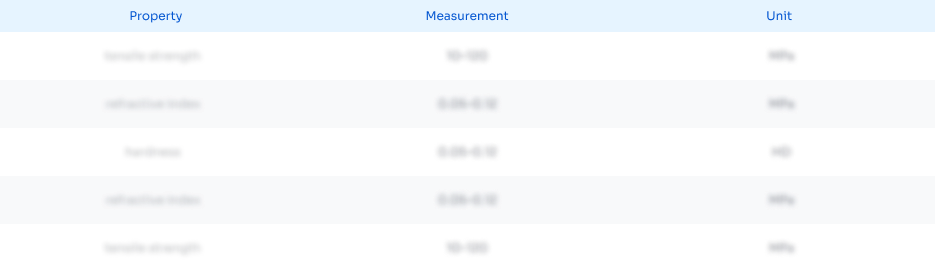
Abstract
Description
Claims
Application Information
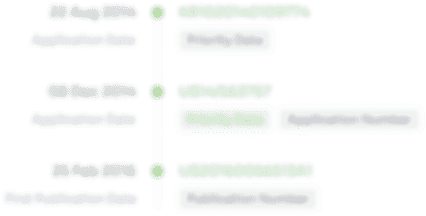
- R&D Engineer
- R&D Manager
- IP Professional
- Industry Leading Data Capabilities
- Powerful AI technology
- Patent DNA Extraction
Browse by: Latest US Patents, China's latest patents, Technical Efficacy Thesaurus, Application Domain, Technology Topic.
© 2024 PatSnap. All rights reserved.Legal|Privacy policy|Modern Slavery Act Transparency Statement|Sitemap