Human motion capture and virtual animation generation method based on deep learning
A human motion and deep learning technology, applied in the computer field, can solve the problems of heavy animation rendering workload, restricting application, and being unsuitable for commercial use on a large scale, so as to improve production efficiency, improve efficiency, and reduce costs.
- Summary
- Abstract
- Description
- Claims
- Application Information
AI Technical Summary
Problems solved by technology
Method used
Image
Examples
Embodiment 1
[0040] Example 1: See Figure 1-8 , a human body motion capture and virtual animation generation method based on deep learning, comprising the following steps:
[0041] A. First, the actor provides the movement posture that needs to be captured by motion, which can be in the form of dance, martial arts, etc.;
[0042] B. The actor motion video data collected by ordinary optical sensing equipment (camera, mobile phone);
[0043] C. Attitude detection network pre-training; the steps of the attitude detection algorithm are as follows: 1. Input the image to be detected into the deep convolutional neural network at different scales, and calculate the response map of each key point; 2. Put each key point in The response graphs at each scale are accumulated to obtain the overall response graph of key points; 3. On the overall response graph of each key point, find the corresponding maximum point and determine the position of the key point. 4. Connect each key point to obtain the in...
Embodiment 2
[0047] Embodiment 2. On the basis of Embodiment 1, the attitude conditional generation confrontation network is composed of three major modules: the attitude detection network P in step B, the generation network G, and the discrimination network D. Among them, the posture detection network P has the same structure and function as in step B, and mainly completes the posture extraction of the avatar with various action postures to obtain posture graphics. The generation network G is composed of a deep convolutional network, and its main function is to complete the automatic creation and rendering of virtual images in a given pose; we use a codec architecture with skip connections, that is, the input of each deconvolution layer is the previous The output of the layer is added to the output of the mirror convolution layer of this layer, so as to ensure that the information of the encoding network can be re-memorized during decoding, so that the generated image retains the details o...
PUM
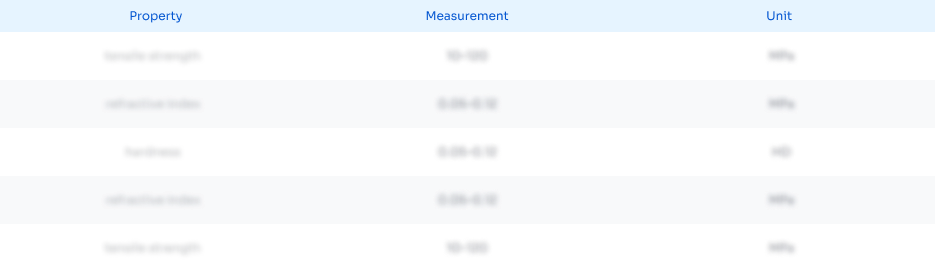
Abstract
Description
Claims
Application Information
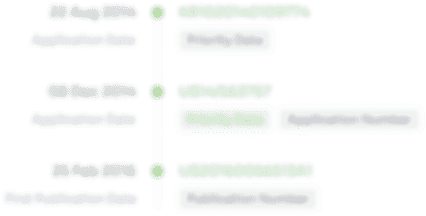
- R&D Engineer
- R&D Manager
- IP Professional
- Industry Leading Data Capabilities
- Powerful AI technology
- Patent DNA Extraction
Browse by: Latest US Patents, China's latest patents, Technical Efficacy Thesaurus, Application Domain, Technology Topic, Popular Technical Reports.
© 2024 PatSnap. All rights reserved.Legal|Privacy policy|Modern Slavery Act Transparency Statement|Sitemap|About US| Contact US: help@patsnap.com