Domain adaptation method based on deep network and confrontation technology
A deep network and domain technology, applied in neural learning methods, biological neural network models, neural architectures, etc., can solve problems such as scarcity of labeled data, achieve easy adaptation, reduce search and control difficulties, strong resistance and public feature expression effect of ability
- Summary
- Abstract
- Description
- Claims
- Application Information
AI Technical Summary
Problems solved by technology
Method used
Image
Examples
Embodiment Construction
[0015] The realization of a domain adaptation method based on deep network and confrontation technology in the present invention consists of an initialization phase, a training phase and a use phase. The initialization phase includes data initialization and model initialization. The training phase includes several gradient backpropagation processes. Depending on the size of the data set, the number of iterations ranges from hundreds to tens of thousands of times until the number of iterations meets a certain condition or the network converges. Then the training is complete.
[0016] Initialization phase:
[0017] Step 1, data initialization. The input to the network is a tensor, usually a color image with three channels of RGB. First, for all images, we scale its size to a tensor of 227×227×3. For grayscale images, simply repeat the image 3 times, it becomes a color map. Secondly, R, G, and B three-dimensional needs to first subtract the mean of the data set, and then divid...
PUM
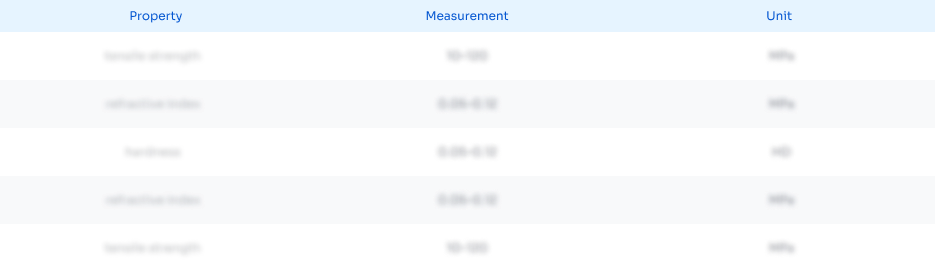
Abstract
Description
Claims
Application Information
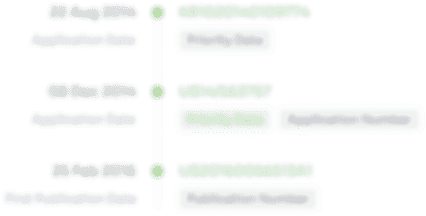
- R&D Engineer
- R&D Manager
- IP Professional
- Industry Leading Data Capabilities
- Powerful AI technology
- Patent DNA Extraction
Browse by: Latest US Patents, China's latest patents, Technical Efficacy Thesaurus, Application Domain, Technology Topic, Popular Technical Reports.
© 2024 PatSnap. All rights reserved.Legal|Privacy policy|Modern Slavery Act Transparency Statement|Sitemap|About US| Contact US: help@patsnap.com