Human face recognition method based on dual data enhancement
A face recognition and data technology, applied in character and pattern recognition, instruments, biological neural network models, etc., can solve problems such as limiting the promotion of face recognition, collecting and organizing data sets, time-consuming and energy-consuming, and limited overall samples.
- Summary
- Abstract
- Description
- Claims
- Application Information
AI Technical Summary
Problems solved by technology
Method used
Image
Examples
Embodiment Construction
[0091] see figure 1 , figure 2 , a face recognition method based on dual data enhancement, comprising the following steps:
[0092] S1. Select the appropriate data set
[0093] After comparison, in the present invention, the open source face data set of the Chinese University of Hong Kong - CelebA is selected as the data source for model training. This data set contains about 200,000 celebrity face images and more than 40 kinds of face feature labels, which lays a data foundation for generating corresponding continuously changing features in the present invention.
[0094] S2, data set preprocessing
[0095] The original size of the image in the CelebA dataset is 178×218, which will bring a large computational burden to the later training, so we crop it to 32×32. At the same time, they are normalized, and the pixel values of the image are unified to [-1,1].
[0096] S3, the first layer of data enhancement
[0097] Build the generation confrontation network model (In...
PUM
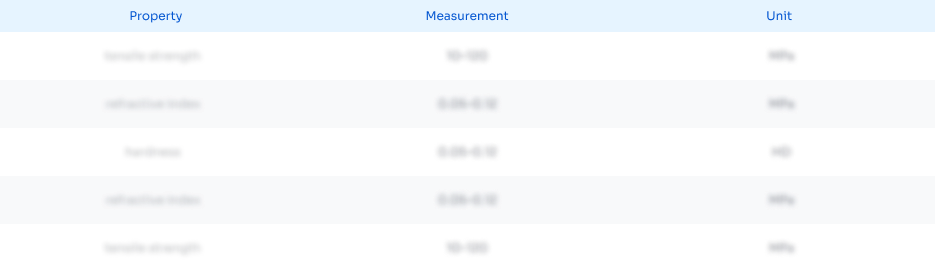
Abstract
Description
Claims
Application Information
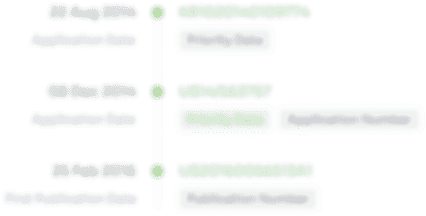
- Generate Ideas
- Intellectual Property
- Life Sciences
- Materials
- Tech Scout
- Unparalleled Data Quality
- Higher Quality Content
- 60% Fewer Hallucinations
Browse by: Latest US Patents, China's latest patents, Technical Efficacy Thesaurus, Application Domain, Technology Topic, Popular Technical Reports.
© 2025 PatSnap. All rights reserved.Legal|Privacy policy|Modern Slavery Act Transparency Statement|Sitemap|About US| Contact US: help@patsnap.com