Digital image automatic labeling method based on uncertainty analysis
A technology of automatic image annotation and digital image, applied in neural learning methods, character and pattern recognition, instruments, etc., can solve uncertainty, cannot accurately estimate the distribution of classes, and the underlying features of the image cannot fully reflect and match the user's retrieval intention. and other problems to achieve the effect of reducing the wrong prediction rate and improving the correct prediction rate
- Summary
- Abstract
- Description
- Claims
- Application Information
AI Technical Summary
Problems solved by technology
Method used
Image
Examples
Embodiment Construction
[0036] The present invention will be further explained below in conjunction with the accompanying drawings and specific embodiments.
[0037] figure 1 It is a business process for implementing an automatic labeling method for digital images based on uncertainty analysis.
[0038] The implementation of the method in this embodiment requires a hardware environment to have a workstation or server that can run deep learning algorithms, and to be equipped with an NVIDIA graphics card. Realize that the language tool used in this embodiment has no special requirement, and C language, C++ language, Python language etc. can realize; There is also no special requirement to operating system platform, Microsoft Windows system, various Linux systems etc. all can be used as operating system operating platform , the invention can be developed into a graphical interface form, and can also be developed into a non-graphical interface form.
[0039] figure 1 It is a functional structural bloc...
PUM
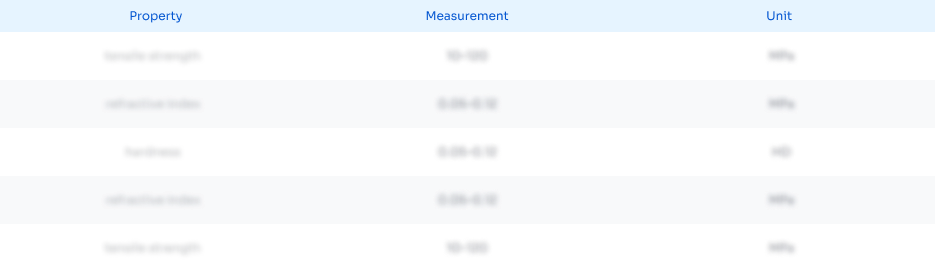
Abstract
Description
Claims
Application Information
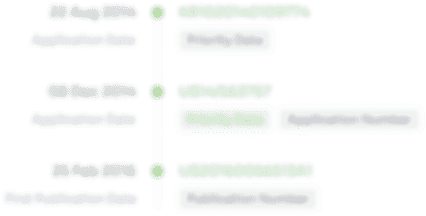
- Generate Ideas
- Intellectual Property
- Life Sciences
- Materials
- Tech Scout
- Unparalleled Data Quality
- Higher Quality Content
- 60% Fewer Hallucinations
Browse by: Latest US Patents, China's latest patents, Technical Efficacy Thesaurus, Application Domain, Technology Topic, Popular Technical Reports.
© 2025 PatSnap. All rights reserved.Legal|Privacy policy|Modern Slavery Act Transparency Statement|Sitemap|About US| Contact US: help@patsnap.com