Image label determination method and device and terminal
A technology for image labeling and determining methods, which is applied in the field of image processing and can solve problems such as poor flexibility of image labeling
- Summary
- Abstract
- Description
- Claims
- Application Information
AI Technical Summary
Problems solved by technology
Method used
Image
Examples
Embodiment 1
[0032] refer to figure 1 , shows a flow chart of steps of a method for determining an image label according to Embodiment 1 of the present invention.
[0033] The image label determination method of the embodiment of the present invention may include the following steps:
[0034] Step 101: input the image into the convolutional neural network, and determine the feature map of the image.
[0035] The image in this embodiment of the present invention may be a single frame image in a video, or just a multimedia image. An image is input into a convolutional neural network, and a feature map is obtained after passing through a convolutional layer or a pooling layer.
[0036] For the specific processing method of inputting the image into the convolutional neural network to obtain the feature map, refer to the existing related technology, which is not specifically limited in the embodiment of the present invention.
[0037] Step 102: Input the feature map into the classifier.
[00...
Embodiment 2
[0048] refer to figure 2 , shows a flow chart of steps of a method for determining an image label according to Embodiment 2 of the present invention.
[0049] The image tag determination method in the embodiment of the present invention may specifically include the following steps:
[0050] Step 201: Construct sample images corresponding to each classification task.
[0051] When constructing sample images corresponding to each classification task, the sample images under each classification task are collected, and each sample image is labeled. Specifically, when labeling sample images, for each sample image, determine the classification task to which the sample image belongs, and the target label corresponding to the sample image under the classification task; set the probability value of the sample image at the target label position to is 1, and the probability value of the sample image at the label position except the target label under each classification task is set to...
Embodiment 3
[0083] refer to image 3 , shows a structural block diagram of an apparatus for determining an image label according to Embodiment 3 of the present invention.
[0084] The image label determination device in the embodiment of the present invention may include: a determination module 301 configured to input an image into a convolutional neural network to determine a feature map of the image; an input module 302 configured to input the feature map into a classification In the device; wherein, the classifier includes a plurality of classification tasks, and each classification task is a multi-label classification task or a single-label classification task; the prediction module 303 is configured to pass each classification task in the classifier, respectively Predict the label corresponding to the feature map; wherein, each label corresponds to a probability value; the label determination module 304 is configured to filter the labels of the image from the predicted labels accordi...
PUM
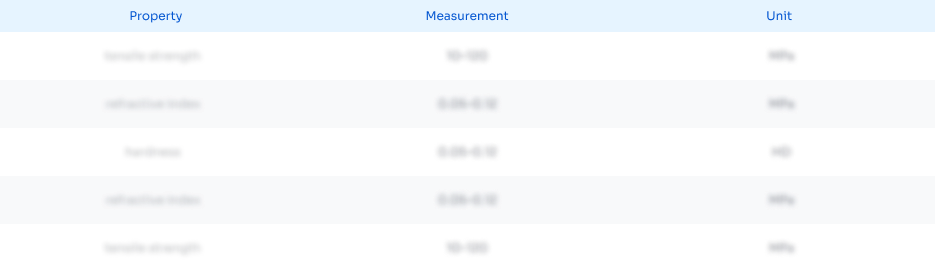
Abstract
Description
Claims
Application Information
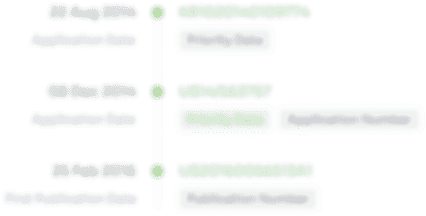
- R&D Engineer
- R&D Manager
- IP Professional
- Industry Leading Data Capabilities
- Powerful AI technology
- Patent DNA Extraction
Browse by: Latest US Patents, China's latest patents, Technical Efficacy Thesaurus, Application Domain, Technology Topic, Popular Technical Reports.
© 2024 PatSnap. All rights reserved.Legal|Privacy policy|Modern Slavery Act Transparency Statement|Sitemap|About US| Contact US: help@patsnap.com