An OFDM detection method based on deep learning to resist inter-subcarrier interference
A technology of inter-subcarrier interference and deep learning, which is applied in phase-modulated carrier systems, modulated carrier systems, baseband systems, etc., to achieve the effect of improving system performance
- Summary
- Abstract
- Description
- Claims
- Application Information
AI Technical Summary
Problems solved by technology
Method used
Image
Examples
example 1
[0067] The simulation conditions are as follows:
[0068] Number of subcarriers 16 Deep Network Layers 30 Modulation QPSK batch size 500 sampling rate 1Mbps The number of channels in the training set 100000 Maximum Doppler Deviation 10kHz overall cycle times 500 Normalized Doppler Offset 0.16
[0069] The simulation channel uses the Rayleigh channel with six delay paths, and the relative power level uses the ITU Vehicle-A channel model parameters. figure 2 The simulation results under the above conditions are given. It can be seen that compared with the direct decorrelation method, the bit error rate of deep network detection is about 2dB lower.
example 2
[0071] The simulation conditions are as follows:
[0072] Number of subcarriers 16 Deep Network Layers 30 Modulation QPSK batch size 500 sampling rate 1Mbps The number of channels in the training set 100000 Maximum Doppler Deviation 15kHz overall cycle times 500 Normalized Doppler Offset 0.24
[0073] The simulation channel uses the Rayleigh channel with six delay paths, and the relative power level uses the ITU Vehicle-A channel model parameters. image 3 The simulation results under the above conditions are given. It can be seen that compared with the direct decorrelation method, the bit error rate of deep network detection is about 4dB lower. and figure 2 In comparison, it can also be seen that the performance of the deep detection network is basically not affected by the size of the Doppler frequency offset.
example 3
[0075] The simulation conditions are as follows:
[0076] Number of subcarriers 32 Deep Network Layers 30 Modulation QPSK batch size 500 sampling rate 1Mbps The number of channels in the training set 100000 Maximum Doppler Deviation 5kHz overall cycle times 500 Normalized Doppler Offset 0.16
[0077] The simulation channel uses Rayleigh channels with four delay paths, and the relative power level uses ITU Pedestrian-A channel model parameters. Figure 4 The simulation results under the above conditions are given. It can be seen that compared with the direct decorrelation method, the bit error rate of deep network detection is about 4-6dB lower.
PUM
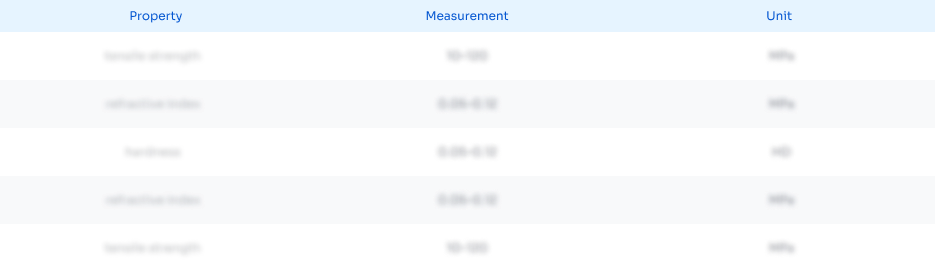
Abstract
Description
Claims
Application Information
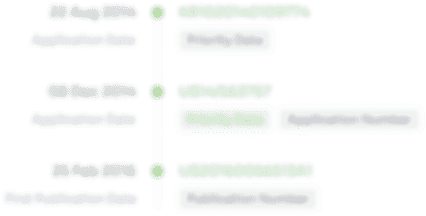
- R&D Engineer
- R&D Manager
- IP Professional
- Industry Leading Data Capabilities
- Powerful AI technology
- Patent DNA Extraction
Browse by: Latest US Patents, China's latest patents, Technical Efficacy Thesaurus, Application Domain, Technology Topic, Popular Technical Reports.
© 2024 PatSnap. All rights reserved.Legal|Privacy policy|Modern Slavery Act Transparency Statement|Sitemap|About US| Contact US: help@patsnap.com