Identification method for potential customers of auto industry based on tri-training
A customer identification and potential technology, which is applied in the field of potential customer identification in the automotive industry, can solve the problems of slow manual identification, poor model generalization ability, and high capital cost, so as to save labor costs and capital costs, improve prediction accuracy, and information Take advantage of the full effect
- Summary
- Abstract
- Description
- Claims
- Application Information
AI Technical Summary
Problems solved by technology
Method used
Image
Examples
Embodiment
[0039] A method for identifying potential customers in the automotive industry based on tri-training, comprising the following steps:
[0040] Step (1): data preprocessing, including the following steps:
[0041] Step (1.1): Abnormal value processing: For each attribute, check whether there is an abnormal attribute value in all data, and delete it if so;
[0042] Step (1.2): Missing value processing: For categorical attributes, the missing value is regarded as a new type; for continuous attributes, an attribute containing missing values is regarded as the dependent variable Y, and other attributes without missing values are regarded as independent variables X, and then use the samples without missing values in Y and the corresponding samples in X as the training set, select the random forest model to train on the training set, and use the trained model to predict the missing values in Y, so as to Fill in the missing values in the original data; do the above processi...
PUM
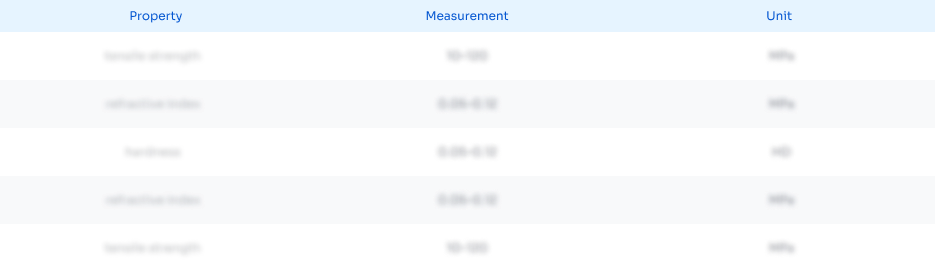
Abstract
Description
Claims
Application Information
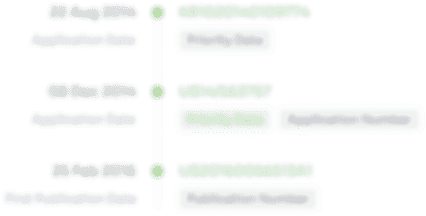
- R&D Engineer
- R&D Manager
- IP Professional
- Industry Leading Data Capabilities
- Powerful AI technology
- Patent DNA Extraction
Browse by: Latest US Patents, China's latest patents, Technical Efficacy Thesaurus, Application Domain, Technology Topic, Popular Technical Reports.
© 2024 PatSnap. All rights reserved.Legal|Privacy policy|Modern Slavery Act Transparency Statement|Sitemap|About US| Contact US: help@patsnap.com