LSTM-based two-dimensional image target class identification method
A technology of two-dimensional images and target categories, applied in the field of image recognition, can solve problems such as low accuracy rate, inability to fully reflect one-dimensional vectors, and inability to fully reflect the two-dimensional topology of image data, etc., to achieve good explainability The effect of improving accuracy
- Summary
- Abstract
- Description
- Claims
- Application Information
AI Technical Summary
Problems solved by technology
Method used
Image
Examples
Embodiment Construction
[0029] The present invention will be further described below in conjunction with the description of the drawings and specific embodiments.
[0030] An LSTM-based two-dimensional image target category identification method, which adds the transformation method when converting a two-dimensional image into a one-dimensional image, according to the circular series connection, row sequence series connection and column sequence connection method from outside to inside respectively. The two-dimensional image is converted into a one-dimensional vector, and then all are sent to the LSTM network for processing (training or identification). Since the LSTM network outputs a result for each conversion method. Then, use a specially designed weighted score fusion scheme to fuse the network output results of different conversion methods, and perform target identification based on the final fusion results. This approach can fully expand the spatial organization of two-bit data into one-bit da...
PUM
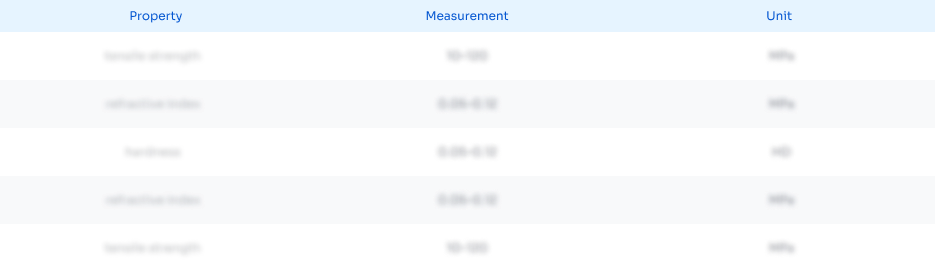
Abstract
Description
Claims
Application Information
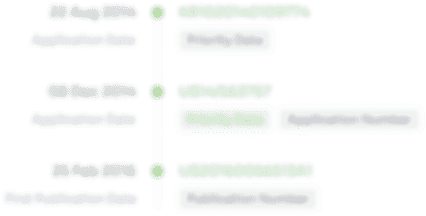
- R&D Engineer
- R&D Manager
- IP Professional
- Industry Leading Data Capabilities
- Powerful AI technology
- Patent DNA Extraction
Browse by: Latest US Patents, China's latest patents, Technical Efficacy Thesaurus, Application Domain, Technology Topic, Popular Technical Reports.
© 2024 PatSnap. All rights reserved.Legal|Privacy policy|Modern Slavery Act Transparency Statement|Sitemap|About US| Contact US: help@patsnap.com