Abnormal detection method of aeroengine gas path based on deep learning and Gaussian distribution
An aero-engine, Gaussian distribution technology, applied in the registration/indication of vehicle operation, registration/indication, instruments, etc., can solve the problems of QAR data not being widely used, high false alarm rate and low accuracy of engine abnormality detection, etc. Achieve the effect of speeding up abnormal detection, distinguishing, and short sampling period
- Summary
- Abstract
- Description
- Claims
- Application Information
AI Technical Summary
Problems solved by technology
Method used
Image
Examples
specific Embodiment approach 1
[0026] Specific implementation mode 1: The specific process of the aero-engine air path anomaly detection method based on deep learning and Gaussian distribution in this embodiment is as follows:
[0027] Step 1. Select a parameter set in the QAR data, and the parameter set includes engine gas path performance parameters and external environment parameters;
[0028] QAR is a quick memory recorder;
[0029] Step 2. Calculate the difference value of the performance parameters of two engines on the same aircraft in the parameter set selected in step 1, and form a new parameter set with the difference value and the external environment parameters as the input of step 3;
[0030] Step 3, select the stacked denoising autoencoder (SDAE) model in the deep learning method to extract data features from the new parameter set in step 2;
[0031] Step 4: Using a Gaussian distribution-based density estimation algorithm to perform anomaly detection on the data features obtained in Step 3 to...
specific Embodiment approach 2
[0032] Embodiment 2: This embodiment differs from Embodiment 1 in that: in the step 1, a parameter set is selected in the QAR data, and the parameter set includes engine air path performance parameters and external environment parameters; the specific process is:
[0033] Select parameter set in QAR data
[0034] Before detecting the abnormality of the gas path of the aero-engine, firstly, according to the structural characteristics and working principle of the aero-engine body, combined with the actual engineering experience, select the parameters that are most closely related to the performance of the gas path of the engine and the most sensitive to the change of the performance of the gas path of the engine to form the engine gas path. Road anomaly detection parameter set.
[0035] The parameter set means:
[0036] S={P 1 ,P 2 ,...P j ,...P k ,P 1 ',P 2 ',...P j ',...P k ',E 1 ,E 2 ,...E q ,...E r}
[0037] In the formula, S is the parameter set selected in th...
specific Embodiment approach 3
[0039] Specific embodiment three: the difference between this embodiment and specific embodiment one or two is: in the described step two, calculate the difference value of two engine performance parameters on the same aircraft in the parameter set selected in step one; The specific process is:
[0040] Δ j =P j '-P j
[0041] In the formula, Δ j is the difference value of the jth gas path performance parameter of the two engines.
[0042] The current research on anomaly detection of air path of aero-engine usually applies anomaly detection algorithm to the operating data of a single engine to identify abnormal points. This has two disadvantages. One is that the gas path performance parameters of the engine will be significantly changed by factors such as operating condition conversion, altitude, temperature, humidity, flight Mach number, etc. This change will lead to the deterioration of the effect of the current anomaly detection method. The second is that each QAR dat...
PUM
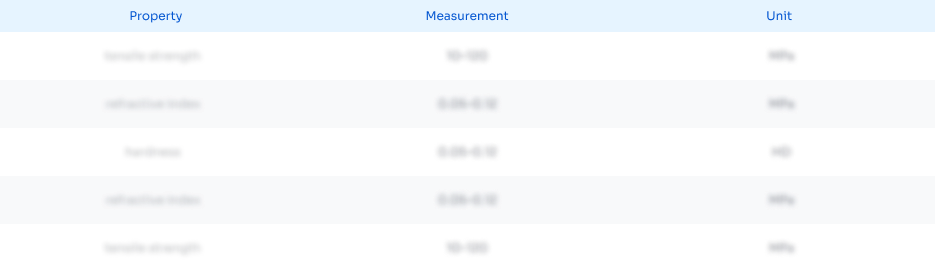
Abstract
Description
Claims
Application Information
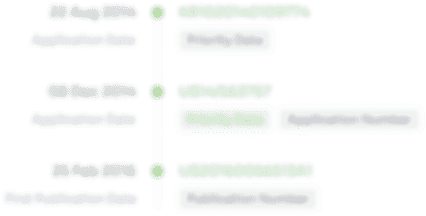
- R&D Engineer
- R&D Manager
- IP Professional
- Industry Leading Data Capabilities
- Powerful AI technology
- Patent DNA Extraction
Browse by: Latest US Patents, China's latest patents, Technical Efficacy Thesaurus, Application Domain, Technology Topic, Popular Technical Reports.
© 2024 PatSnap. All rights reserved.Legal|Privacy policy|Modern Slavery Act Transparency Statement|Sitemap|About US| Contact US: help@patsnap.com