Multilayer perceptron artificial nerve network based on residual error network
A technology of artificial neural network and multi-layer perceptron, applied in the field of bionic network computing, can solve problems such as weak effects, achieve the effect of narrow application range, speed up the training process, and reduce the amount of calculation
- Summary
- Abstract
- Description
- Claims
- Application Information
AI Technical Summary
Problems solved by technology
Method used
Image
Examples
Embodiment
[0028] This embodiment discloses a multi-layer perceptron artificial neural network based on the residual network, which solves the degradation problem of traditional neural networks. Traditional neural networks are usually directly transmitted, including multi-layer perceptrons, convolutional neural networks The internet.
[0029] The traditional multi-layer perceptron consists of one or more hidden layers, each hidden layer contains multiple neurons, assuming that the input of neurons in the i-th layer is net i,j , the output is s i,j , then one can get
[0030]
[0031] the s i,j =f(net i,j )(6)
[0032] Among them, n is the number of neurons directly connected to the neuron in the previous layer, and the constant b i,j represents the input bias. We endow each layer with linear or non-linear features through the activation function f. For the output layer, our predicted result is f(x (i) ;W), the target label is y (i) , if the sum of squared errors is used to ob...
PUM
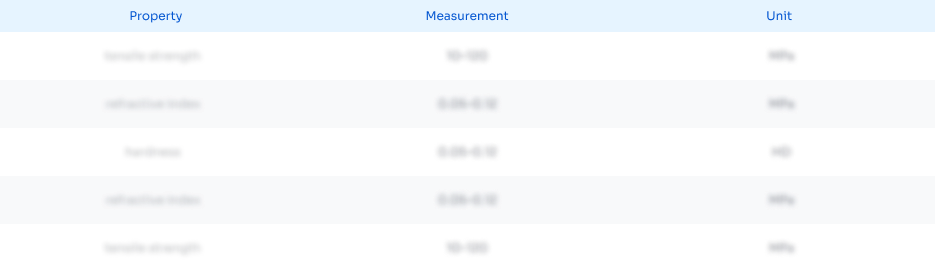
Abstract
Description
Claims
Application Information
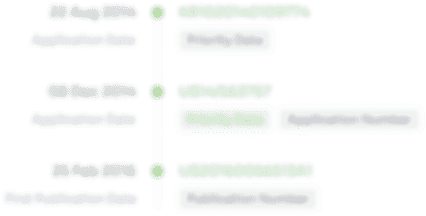
- R&D Engineer
- R&D Manager
- IP Professional
- Industry Leading Data Capabilities
- Powerful AI technology
- Patent DNA Extraction
Browse by: Latest US Patents, China's latest patents, Technical Efficacy Thesaurus, Application Domain, Technology Topic.
© 2024 PatSnap. All rights reserved.Legal|Privacy policy|Modern Slavery Act Transparency Statement|Sitemap