Fast traffic signboard recognition method based on convolution neural network
A technology of convolutional neural network and traffic signs, applied in neural learning methods, biological neural network models, neural architectures, etc., can solve problems such as single classification, and achieve high recognition rate, fast calculation speed, and fast speed
- Summary
- Abstract
- Description
- Claims
- Application Information
AI Technical Summary
Problems solved by technology
Method used
Image
Examples
Embodiment Construction
[0024] In order to enable your examiners to further understand the structure, features and other purposes of the present invention, the attached preferred embodiments are now described in detail as follows. The described preferred embodiments are only used to illustrate the technical solutions of the present invention, not to limit the present invention. invention.
[0025] Process flow of the present invention such as figure 1 As shown, first, according to the situation in the actual recognition process, the training sample data set after augmentation and optimization is obtained through image transformation; then, the FTSR-CNN network structure is designed and the FTSR-CNN network model is trained; finally, the network model is applied Fast or real-time identification of traffic signs is completed; the specific implementation process of the technical solution of the present invention will be described below in conjunction with the accompanying drawings.
[0026] 1. Prepare...
PUM
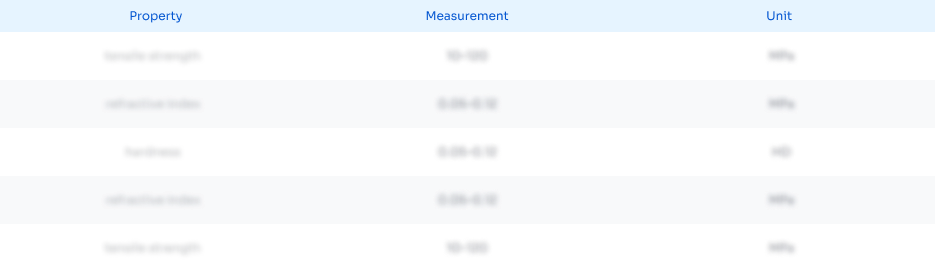
Abstract
Description
Claims
Application Information
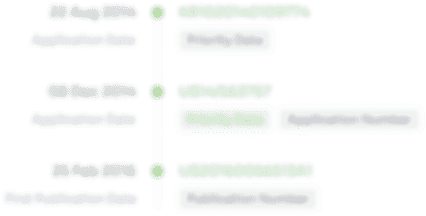
- R&D Engineer
- R&D Manager
- IP Professional
- Industry Leading Data Capabilities
- Powerful AI technology
- Patent DNA Extraction
Browse by: Latest US Patents, China's latest patents, Technical Efficacy Thesaurus, Application Domain, Technology Topic, Popular Technical Reports.
© 2024 PatSnap. All rights reserved.Legal|Privacy policy|Modern Slavery Act Transparency Statement|Sitemap|About US| Contact US: help@patsnap.com