Image classification method based on high-constraint high-dispersion principal component analysis network
A principal component analysis and classification method technology, applied in the field of image classification based on highly constrained and highly dispersed principal component analysis network, can solve problems such as computational complexity and time complexity
- Summary
- Abstract
- Description
- Claims
- Application Information
AI Technical Summary
Problems solved by technology
Method used
Image
Examples
Embodiment Construction
[0038] The present invention will be further described in detail in conjunction with the following specific embodiments and accompanying drawings. The process, conditions, experimental methods, etc. for implementing the present invention, except for the content specifically mentioned below, are common knowledge and common knowledge in this field, and the present invention has no special limitation content.
[0039] Such as figure 1 As shown, the image classification method based on the highly constrained and highly dispersed principal component analysis network of the present invention, the input image passes through at least one set of convolutional layers and nonlinear transformation layers, and a feature pooling layer, which specifically includes the following steps:
[0040] Convolution and nonlinear change step: in the convolution layer, a plurality of convolution kernels for each stage feature extraction are learned from the training set by PCA; in the nonlinear transfor...
PUM
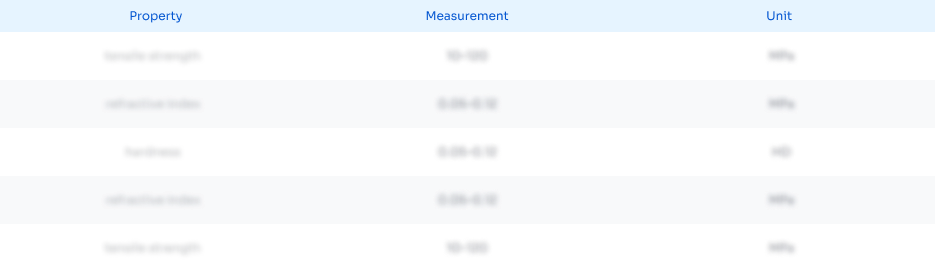
Abstract
Description
Claims
Application Information
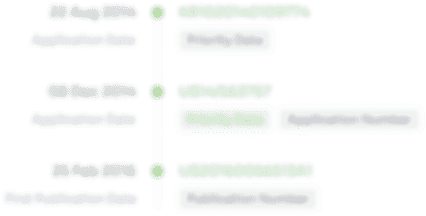
- R&D Engineer
- R&D Manager
- IP Professional
- Industry Leading Data Capabilities
- Powerful AI technology
- Patent DNA Extraction
Browse by: Latest US Patents, China's latest patents, Technical Efficacy Thesaurus, Application Domain, Technology Topic, Popular Technical Reports.
© 2024 PatSnap. All rights reserved.Legal|Privacy policy|Modern Slavery Act Transparency Statement|Sitemap|About US| Contact US: help@patsnap.com