Multi-data-stream anomaly detection method based on context
A multi-data stream, anomaly detection technology, applied in the field of anomaly detection of historical behavior information, can solve the problems of difficult threshold setting, no consideration, impractical real-time detection, etc., to achieve low time complexity, reduce difficulty, and improve detection accuracy Effect
- Summary
- Abstract
- Description
- Claims
- Application Information
AI Technical Summary
Problems solved by technology
Method used
Image
Examples
Embodiment Construction
[0023] combine figure 1 , a context-based multi-stream anomaly detection method, comprising the following steps:
[0024] Step 1, multi-stream acquisition and snapshot generation, the process is as follows:
[0025] Step 1.1, given a distributed computing system composed of n isomorphic computing nodes;
[0026] Step 1.2, synchronously record the observation value of the i-th computing node at time t in Indicates the component of the i-th computing node on the m-th observation dimension of the observed value at time t, 1≤i≤n, each component represents a node metric of interest, and the metrics of isomorphic computing nodes are exactly the same , the value of m is determined by the total number of metrics;
[0027] Step 1.3, constitute the data flow S corresponding to the computing node i i ={s i1 ,s i2 ,s i3 ,...,s it}, S i is an ordered but infinite sequence of data observations;
[0028] Step 1.4, the data streams of n computing nodes form a data stream set S={...
PUM
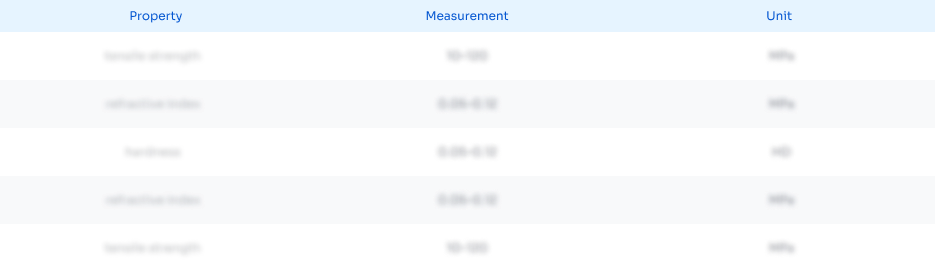
Abstract
Description
Claims
Application Information
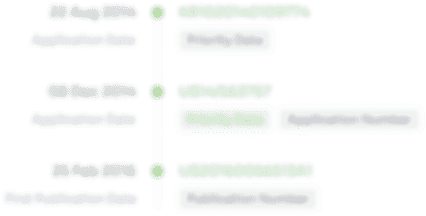
- Generate Ideas
- Intellectual Property
- Life Sciences
- Materials
- Tech Scout
- Unparalleled Data Quality
- Higher Quality Content
- 60% Fewer Hallucinations
Browse by: Latest US Patents, China's latest patents, Technical Efficacy Thesaurus, Application Domain, Technology Topic, Popular Technical Reports.
© 2025 PatSnap. All rights reserved.Legal|Privacy policy|Modern Slavery Act Transparency Statement|Sitemap|About US| Contact US: help@patsnap.com