Internet of Things intrusion detection method based on self-supervised learning and self-knowledge distillation
An intrusion detection and supervised learning technology, applied in the field of Internet of Things, can solve the problems of time-consuming, expensive acquisition of attack tag data, and few Internet of Things intrusion detection systems, etc., to improve the detection speed, generalization ability and representation learning. ability to avoid the effect of over-reliance on label data
- Summary
- Abstract
- Description
- Claims
- Application Information
AI Technical Summary
Problems solved by technology
Method used
Image
Examples
Embodiment Construction
[0040] The following is a specific specific embodiment to illustrate the implementation of the present invention. Those who are familiar with this technology can easily understand the other advantages and effects of the present invention exposed by this technology. Obvious , Not all embodiments. Based on the embodiments in the present invention, all other embodiments obtained by ordinary technical personnel in the art under the premise of not creating creative labor belong to the protection of the present invention.
[0041] Assist figure 1 The specific steps of the invention use the lightweight intrusive detection model for the invasion detection are as follows:
[0042] (1) Data pre -processing the intrusion detection dataset, which includes character data thermal coding and data home -to -one processing of data pre -processing;
[0043] (2) Lightweight invasion detection model first stage training:
[0044] (21) Determine the network structure of the online network and the target...
PUM
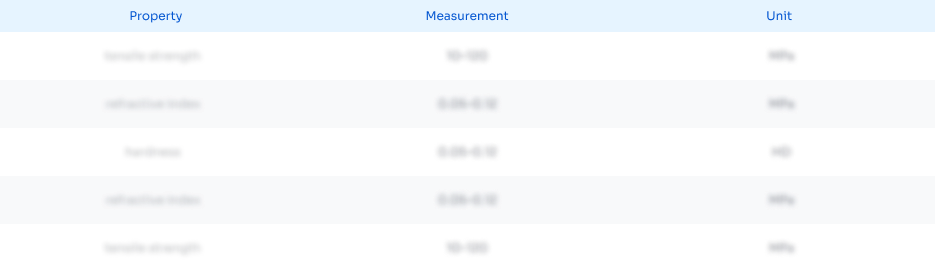
Abstract
Description
Claims
Application Information
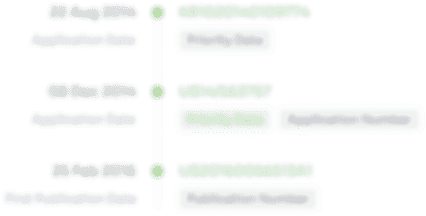
- R&D Engineer
- R&D Manager
- IP Professional
- Industry Leading Data Capabilities
- Powerful AI technology
- Patent DNA Extraction
Browse by: Latest US Patents, China's latest patents, Technical Efficacy Thesaurus, Application Domain, Technology Topic, Popular Technical Reports.
© 2024 PatSnap. All rights reserved.Legal|Privacy policy|Modern Slavery Act Transparency Statement|Sitemap|About US| Contact US: help@patsnap.com