Security calculation method based on joint learning
A computing method and security technology, applied in the field of federated learning, can solve the problems of data leakage, undiscovered federated learning, technical irregularity, etc., and achieve the effects of high security value, rich statistical accuracy, and improved versatility
- Summary
- Abstract
- Description
- Claims
- Application Information
AI Technical Summary
Problems solved by technology
Method used
Image
Examples
Embodiment 1
[0058] see image 3 , the security computing method based on joint learning of the present invention is applied to the data set enhancement training in joint learning, which comprises the following steps:
[0059] Step 1: Establish a joint learning model: it consists of an encoder, a communication unit, an integration unit and a classifier.
[0060] Step 2: For each object participating in the joint participation, there is a certain number of samples, and the number of samples is received, stored, and classified into the database through the encoder, communication unit and classifier. The data is integrated in the database according to the data characteristics and marked as the first characteristic data, the second characteristic data, the Nth characteristic data;
[0061] Step 3: According to the joint training task, preprocess the input task information, identify the candidate values according to the regular expression related to the task value, use the value as the trigg...
Embodiment 2
[0066] see Figure 4 , applying the joint learning-based security computing method of the present invention to the feature aggregation of the data set in joint learning, which includes the following steps:
[0067] Step 1: Establish a joint learning model: it consists of an encoder, a communication unit, an integration unit and a classifier.
[0068] Step 2: For each object participating in the joint participation, there is a certain number of samples, and the number of samples is received, stored, and classified into the database through the encoder, communication unit and classifier. The data is integrated in the database according to the data characteristics and marked as the first characteristic data, the second characteristic data, the Nth characteristic data;
[0069] Step 3: According to the joint training task, preprocess the input task information, identify the candidate values according to the regular expression related to the task value, use the value as the trig...
Embodiment 3
[0074] see Figure 5 , applying the joint learning-based security computing method of the present invention to the data labeling in joint learning, which comprises the following steps:
[0075] The first step: establish a joint learning model: it consists of an encoder, a communication unit, an integration unit and a classifier.
[0076] Step 2: Each object participating in the joint has a certain number of samples, some have characteristic data samples, some have labeled data samples, and the number of samples is received, stored, and classified through the encoder, communication unit and classifier. The corresponding data is stored in the database, and the integration unit integrates and marks the data received in real time in the database according to the data characteristics as the first characteristic data, the second characteristic data, the Nth characteristic data;
[0077] Step 3: According to the joint training task, preprocess the input task information, identify the ...
PUM
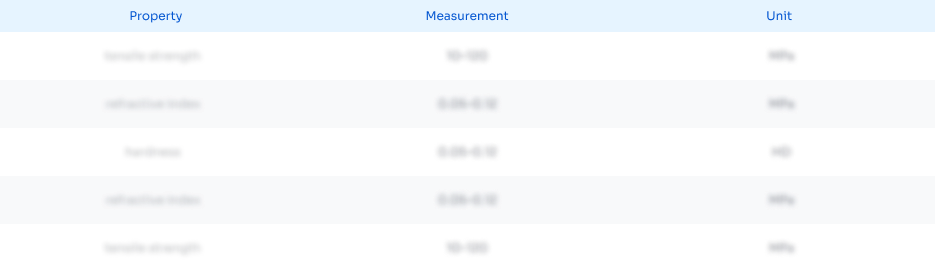
Abstract
Description
Claims
Application Information
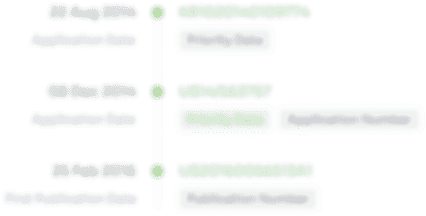
- R&D Engineer
- R&D Manager
- IP Professional
- Industry Leading Data Capabilities
- Powerful AI technology
- Patent DNA Extraction
Browse by: Latest US Patents, China's latest patents, Technical Efficacy Thesaurus, Application Domain, Technology Topic, Popular Technical Reports.
© 2024 PatSnap. All rights reserved.Legal|Privacy policy|Modern Slavery Act Transparency Statement|Sitemap|About US| Contact US: help@patsnap.com