Non-intrusive load decomposition method based on seq2point network
A load-decomposing, non-invasive technology, applied in neural learning methods, biological neural network models, character and pattern recognition, etc., can solve problems such as inappropriateness, abnormal data collection, and low recognition accuracy, and reduce recognition errors , Simple network structure, high resolution effect
- Summary
- Abstract
- Description
- Claims
- Application Information
AI Technical Summary
Problems solved by technology
Method used
Image
Examples
Embodiment Construction
[0034] The present application will be further described below with reference to the accompanying drawings. The following examples are only used to more clearly illustrate the technical solutions of the present invention, and cannot be used to limit the protection scope of the present application.
[0035] Non-intrusive load identification is of great significance to power dispatching and risk estimation of power grid systems. Aiming at the low recognition accuracy and high computational cost of the current non-intrusive load decomposition algorithm, the present invention proposes a non-intrusive load decomposition algorithm based on seq2point network, a sequence-to-point deep learning model based on convolutional neural network, the The model can have better load identification ability for household appliances under the low frequency sampling of 1Hz and below. Using public low-frequency datasets to train and test the model, and comparing with existing algorithms, it is found...
PUM
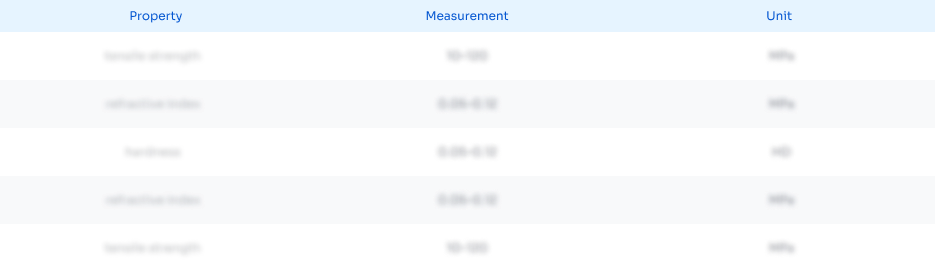
Abstract
Description
Claims
Application Information
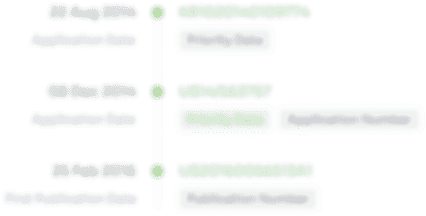
- R&D Engineer
- R&D Manager
- IP Professional
- Industry Leading Data Capabilities
- Powerful AI technology
- Patent DNA Extraction
Browse by: Latest US Patents, China's latest patents, Technical Efficacy Thesaurus, Application Domain, Technology Topic, Popular Technical Reports.
© 2024 PatSnap. All rights reserved.Legal|Privacy policy|Modern Slavery Act Transparency Statement|Sitemap|About US| Contact US: help@patsnap.com