BM-CNN model-based dangerous action identification method and system in short video APP
An action recognition, short video technology, applied in the field of dangerous action recognition and system in short video APP based on BM-CNN model, can solve the problems of supervision lag, manual review, low accuracy and efficiency, and make up for the loss of speed , to ensure security and stability, full-featured effects
- Summary
- Abstract
- Description
- Claims
- Application Information
AI Technical Summary
Problems solved by technology
Method used
Image
Examples
Embodiment 1
[0089] According to a kind of dangerous action recognition system in short video APP based on BM-CNN model provided by the present invention, such as Figure 1-Figure 7 shown, including:
[0090] Initialization module: acquire video and preprocess the video and process it with convolutional neural network, it is automatically called when the system starts to run, and can be called again by the administrator module;
[0091] Administrator module: process the data set and conduct manual review, the module is called by the administrator, and the function is displayed to the user;
[0092] User module: provide the function of uploading video and result display, and interact with the work module to realize the function of action recognition and classification;
[0093] Working module: Receive video, realize action recognition and classification, and can expand the data set of different classified actions.
[0094] Specifically, in the initialization module:
[0095] The workflow...
Embodiment 2
[0146] Embodiment 2 is a preferred example of Embodiment 1, in order to describe the present invention in more detail.
[0147] The invention relates to the intersection of digital video processing technology, artificial intelligence and pattern recognition technology. The invention relates to a method and system for identifying dangerous actions in a short video APP based on a BM-CNN (Boundary-Matching Convolutional Neural Network) model. Here's a video of the dangerous action. The method first preprocesses the input video, and then processes the original video and inputs it into an improved three-dimensional convolutional neural network, and finally obtains the judgment result, which is used to distinguish the video of normal behavior and dangerous behavior.
[0148] The system can be divided into different modules for different objects, including: initialization module, work module, administrator module and user module. in:
[0149] The initialization module is a module ...
PUM
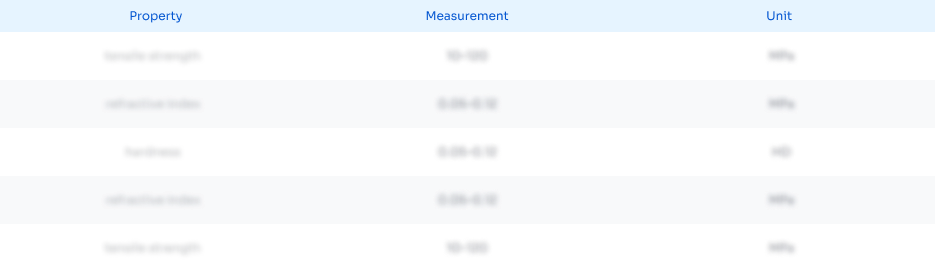
Abstract
Description
Claims
Application Information
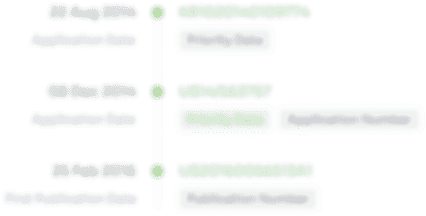
- R&D Engineer
- R&D Manager
- IP Professional
- Industry Leading Data Capabilities
- Powerful AI technology
- Patent DNA Extraction
Browse by: Latest US Patents, China's latest patents, Technical Efficacy Thesaurus, Application Domain, Technology Topic, Popular Technical Reports.
© 2024 PatSnap. All rights reserved.Legal|Privacy policy|Modern Slavery Act Transparency Statement|Sitemap|About US| Contact US: help@patsnap.com