Medical image segmentation task lightweight system construction method
A technology of medical images and construction methods, applied in the field of deep learning, to alleviate the data distribution gap, improve the training speed, and alleviate the effect of overfitting problems
- Summary
- Abstract
- Description
- Claims
- Application Information
AI Technical Summary
Problems solved by technology
Method used
Image
Examples
Embodiment 1
[0037] In Embodiment 1 of the present invention, the method of the present invention is described through the aneurysm segmentation task by taking the construction of a 3D CNN lightweight segmentation system as an example. with X∈R w×h×d and Y∈R w×h×d represent the original medical volume data and its corresponding annotation results, respectively, and w, h, and d represent the number of slices observed from the sagittal, coronal, and transverse planes, respectively.
[0038] 1. Sample preprocessing
[0039] The method of thresholding is used to remove some unrelated tissues in the original medical image, and the ability of the network to extract the aneurysm features is improved. The present invention first normalizes the HU value of the original image to the range of [1800, 2300], and then Normalized to [0, 1].
[0040] 2. Training sample generation
[0041] Due to the limitation of video memory, it is difficult to directly train and test 3D CNNs at the original resoluti...
PUM
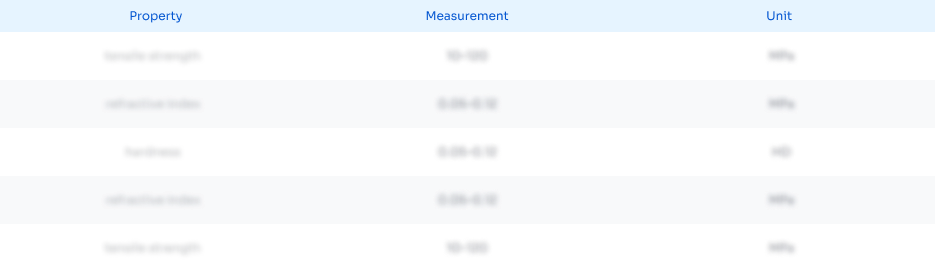
Abstract
Description
Claims
Application Information
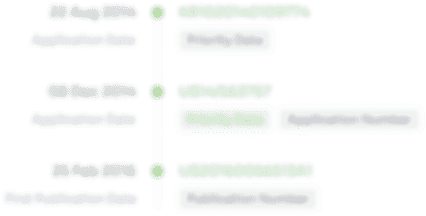
- R&D Engineer
- R&D Manager
- IP Professional
- Industry Leading Data Capabilities
- Powerful AI technology
- Patent DNA Extraction
Browse by: Latest US Patents, China's latest patents, Technical Efficacy Thesaurus, Application Domain, Technology Topic, Popular Technical Reports.
© 2024 PatSnap. All rights reserved.Legal|Privacy policy|Modern Slavery Act Transparency Statement|Sitemap|About US| Contact US: help@patsnap.com