Three-dimensional semantic segmentation method based on channel attention and multi-scale fusion
A multi-scale fusion and semantic segmentation technology, which is applied in neural learning methods, instruments, biological neural network models, etc., can solve problems such as information loss, inability to make full use of 3D data, and result impact, and achieve the effect of improving segmentation results
- Summary
- Abstract
- Description
- Claims
- Application Information
AI Technical Summary
Problems solved by technology
Method used
Image
Examples
Embodiment
[0085] The dataset used in this example is the S3DIS dataset, which is collected from the indoor environments of three different buildings and contains 271 rooms in 6 areas. It has a total of 695,878,620 point clouds, each of which has corresponding coordinates and color information, as well as semantic labels such as chairs, tables, floors, walls, etc., with a total of 13 categories. In this embodiment, regions 1, 2, 3, 4, and 6 are selected for training, and region 5 is selected for testing. During training, this embodiment samples the input points into a uniform number of 4096 points, and uses all the points during testing.
[0086] This example trains 150 epochs on two GeForce RTX 2080Ti GPUs with a batch size of 16, using an SGD optimizer with an initial learning rate of 0.05, momentum of 0.9, and weight decay rate of 10 -4 , and implemented on the Pytorch platform using Linux. After using the training set to train the network to obtain the model, the model performance ...
PUM
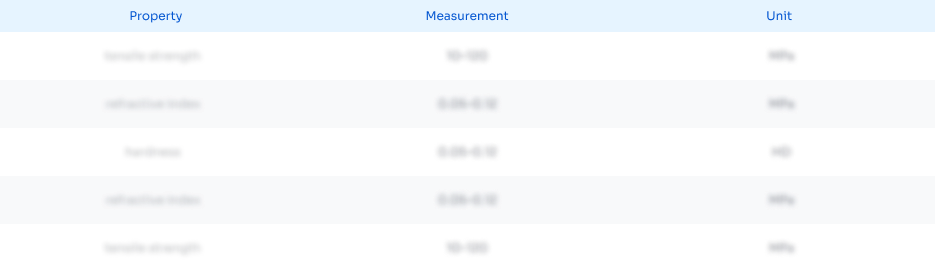
Abstract
Description
Claims
Application Information
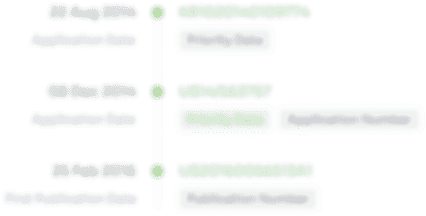
- Generate Ideas
- Intellectual Property
- Life Sciences
- Materials
- Tech Scout
- Unparalleled Data Quality
- Higher Quality Content
- 60% Fewer Hallucinations
Browse by: Latest US Patents, China's latest patents, Technical Efficacy Thesaurus, Application Domain, Technology Topic, Popular Technical Reports.
© 2025 PatSnap. All rights reserved.Legal|Privacy policy|Modern Slavery Act Transparency Statement|Sitemap|About US| Contact US: help@patsnap.com